Filtering by
- Creators: Wang, Wen-Xu
Two classes of scaling behaviours, namely the super-linear scaling of links or activities, and the sub-linear scaling of area, diversity, or time elapsed with respect to size have been found to prevail in the growth of complex networked systems. Despite some pioneering modelling approaches proposed for specific systems, whether there exists some general mechanisms that account for the origins of such scaling behaviours in different contexts, especially in socioeconomic systems, remains an open question. We address this problem by introducing a geometric network model without free parameter, finding that both super-linear and sub-linear scaling behaviours can be simultaneously reproduced and that the scaling exponents are exclusively determined by the dimension of the Euclidean space in which the network is embedded. We implement some realistic extensions to the basic model to offer more accurate predictions for cities of various scaling behaviours and the Zipf distribution reported in the literature and observed in our empirical studies. All of the empirical results can be precisely recovered by our model with analytical predictions of all major properties. By virtue of these general findings concerning scaling behaviour, our models with simple mechanisms gain new insights into the evolution and development of complex networked systems.
In 2014/2015, Arizona State University (ASU) Libraries, the Labriola National American Indian Data Center, and the ASU American Indian Studies Department completed an ASU Institute for Humanities Research (IHR) seed grant entitled “Carlos Montezuma’s Wassaja Newsletter: Digitization, Access and Context” to digitize all ASU held issues of the newsletter Wassaja Freedom’s Signal for the Indian, which Yavapai activist-intellectual Carlos Montezuma, MD (1866-1923) self-published during 1916-1922. The grant team additionally selected a portion of the ASU Libraries Carlos Montezuma archival collection for digitization to provide a more complete picture of Dr. Carlos Montezuma’s life and work.
The ASU grant team produced a searchable online collection on the ASU Digital Repository and created an online exhibition in conjunction with the IHR Nexus Lab’s Developing Wassaja Project. The Nexus Lab’s role at ASU is to grow the digital humanities through interdisciplinary collaborations bringing together humanities, science, and technology. The Nexus Lab partnered with the grant team to create the Developing Wassaja Project which provided an opportunity for faculty, staff, and students at ASU to engage in electronic publication through web application development.
The resulting web platform, Wassaja: A Carlos Montezuma Project, provides context for this digitized collection and facilitates community interaction, including a partnership with Dr. Montezuma’s home community the Fort McDowell Yavapai Nation. In this webcast, Digital Projects Librarian Matthew Harp, Developing Wassaja Project team member Joe Buenker (subject librarian), and grant team member Joyce Martin (librarian and curator of the Labriola National American Indian Data Center) will discuss and demonstrate the resources created and the resulting partnership with the Fort McDowell Yavapai Nation. The webcast will focus on identifying collaborators and needed skills to engage in Digital Humanities research and on identifying the stages of a collaborative project.
Participants will gain insight on working directly with diverse communities; overcoming technical limitations of traditional institutional repositories; collaborative strategies with faculty, research centers, and cultural heritage societies; solutions for moving hidden collections into an engaging digital exhibition; integrating digital humanities research and instruction with library curation; and preparing for long term costs and management issues.
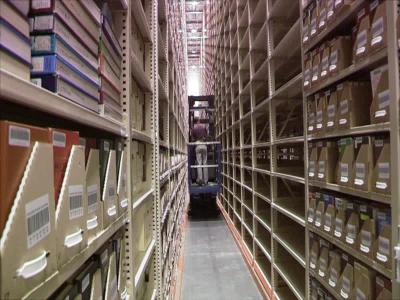
Anthropology librarian Juliann Couture and Joyce Martin, curator of the Labriola National American Indian Data Center, looking at the Center's display of unique Hopi Kachina dolls. Four of the kachinas (Navan Kachina; Talavi Kachina; Flute Kachina; and Ahöla Kachina) were created by artist, carver, and former ASU employee Tony Dukepoo as a gift to the libraries in 1979. The kachina dolls are on display in the Labriola Center located on the 2nd floor of the Hayden Library on ASU's Tempe campus.
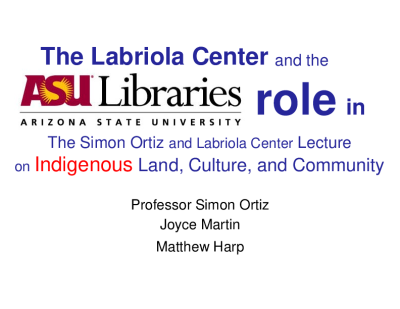
The Simon Ortiz and Labriola Center Lecture on Indigenous Land, Culture, and Community addresses topics and issues across disciplines in the arts, humanities, sciences, and politics. Underscoring Indigenous American experiences and perspectives, this Series seeks to create and celebrate knowledge that evolves from an Indigenous worldview that is inclusive and that is applicable to all walks of life.” Professor Simon Ortiz discussed the overall nature of the Series, especially emphasizing the global nature of Indigenous concerns. Joyce Martin and Matthew Harp elaborated on the contributions of the Labriola National American Indian Data Center and ASU Libraries to the Series.
The Labriola Center hosts an informal event in Hayden Library which facilitates close interaction between the featured speaker and audience members. The ASU Libraries records the evening lectures which take place at the Heard Museum and presents both an audio podcast and streaming video of each lecture on the ASU Library Channel webpage. This lecture series provides not only a chance for community discussion at the events themselves, but through the innovative use of technology the ASU Libraries enables additional forums for discussion in blogs and web pages which choose to link to the streaming videos.
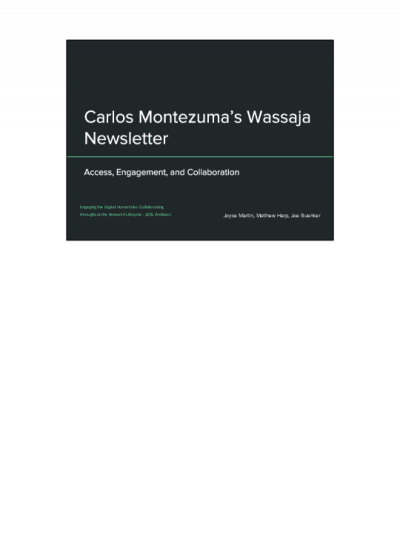
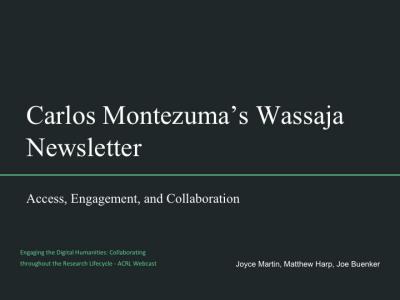

Given a complex geospatial network with nodes distributed in a two-dimensional region of physical space, can the locations of the nodes be determined and their connection patterns be uncovered based solely on data? We consider the realistic situation where time series/signals can be collected from a single location. A key challenge is that the signals collected are necessarily time delayed, due to the varying physical distances from the nodes to the data collection centre. To meet this challenge, we develop a compressive-sensing-based approach enabling reconstruction of the full topology of the underlying geospatial network and more importantly, accurate estimate of the time delays. A standard triangularization algorithm can then be employed to find the physical locations of the nodes in the network. We further demonstrate successful detection of a hidden node (or a hidden source or threat), from which no signal can be obtained, through accurate detection of all its neighbouring nodes. As a geospatial network has the feature that a node tends to connect with geophysically nearby nodes, the localized region that contains the hidden node can be identified.

Recent works revealed that the energy required to control a complex network depends on the number of driving signals and the energy distribution follows an algebraic scaling law. If one implements control using a small number of drivers, e.g. as determined by the structural controllability theory, there is a high probability that the energy will diverge. We develop a physical theory to explain the scaling behaviour through identification of the fundamental structural elements, the longest control chains (LCCs), that dominate the control energy. Based on the LCCs, we articulate a strategy to drastically reduce the control energy (e.g. in a large number of real-world networks). Owing to their structural nature, the LCCs may shed light on energy issues associated with control of nonlinear dynamical networks.

A challenging problem in network science is to control complex networks. In existing frameworks of structural or exact controllability, the ability to steer a complex network toward any desired state is measured by the minimum number of required driver nodes. However, if we implement actual control by imposing input signals on the minimum set of driver nodes, an unexpected phenomenon arises: due to computational or experimental error there is a great probability that convergence to the final state cannot be achieved. In fact, the associated control cost can become unbearably large, effectively preventing actual control from being realized physically. The difficulty is particularly severe when the network is deemed controllable with a small number of drivers. Here we develop a physical controllability framework based on the probability of achieving actual control. Using a recently identified fundamental chain structure underlying the control energy, we offer strategies to turn physically uncontrollable networks into physically controllable ones by imposing slightly augmented set of input signals on properly chosen nodes. Our findings indicate that, although full control can be theoretically guaranteed by the prevailing structural controllability theory, it is necessary to balance the number of driver nodes and control cost to achieve physical control.

Network reconstruction is a fundamental problem for understanding many complex systems with unknown interaction structures. In many complex systems, there are indirect interactions between two individuals without immediate connection but with common neighbors. Despite recent advances in network reconstruction, we continue to lack an approach for reconstructing complex networks with indirect interactions. Here we introduce a two-step strategy to resolve the reconstruction problem, where in the first step, we recover both direct and indirect interactions by employing the Lasso to solve a sparse signal reconstruction problem, and in the second step, we use matrix transformation and optimization to distinguish between direct and indirect interactions. The network structure corresponding to direct interactions can be fully uncovered. We exploit the public goods game occurring on complex networks as a paradigm for characterizing indirect interactions and test our reconstruction approach. We find that high reconstruction accuracy can be achieved for both homogeneous and heterogeneous networks, and a number of empirical networks in spite of insufficient data measurement contaminated by noise. Although a general framework for reconstructing complex networks with arbitrary types of indirect interactions is yet lacking, our approach opens new routes to separate direct and indirect interactions in a representative complex system.