
Adaptive comanagement endeavors to increase knowledge and responsiveness in the face of uncertainty and complexity. However, when collaboration between agency and nonagency stakeholders is mandated, rigid institutions may hinder participation and ecological outcomes. In this case study we analyzed qualitative data to understand how participants perceive strengths and challenges within an emerging adaptive comanagement in the Agua Fria Watershed in Arizona, USA that utilizes insight and personnel from a long-enduring comanagement project, Las Cienegas. Our work demonstrates that general lessons and approaches from one project may be transferable, but particular institutions, management structures, or projects must be place-specific. As public agencies establish and expand governance networks throughout the western United States, our case study has shed light on how to maintain a shared vision and momentum within an inherently murky and shared decision-making environment.


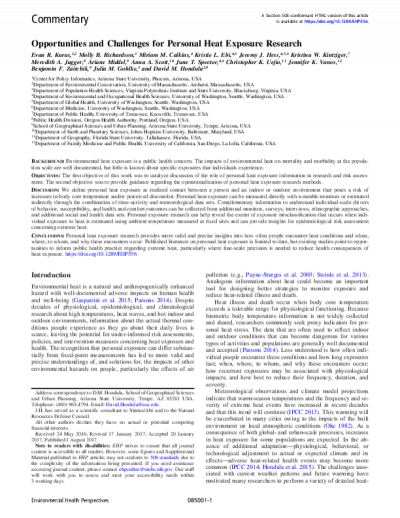
Background:
Environmental heat exposure is a public health concern. The impacts of environmental heat on mortality and morbidity at the population scale are well documented, but little is known about specific exposures that individuals experience.
Objectives:
The first objective of this work was to catalyze discussion of the role of personal heat exposure information in research and risk assessment. The second objective was to provide guidance regarding the operationalization of personal heat exposure research methods.
Discussion:
We define personal heat exposure as realized contact between a person and an indoor or outdoor environment that poses a risk of increases in body core temperature and/or perceived discomfort. Personal heat exposure can be measured directly with wearable monitors or estimated indirectly through the combination of time–activity and meteorological data sets. Complementary information to understand individual-scale drivers of behavior, susceptibility, and health and comfort outcomes can be collected from additional monitors, surveys, interviews, ethnographic approaches, and additional social and health data sets. Personal exposure research can help reveal the extent of exposure misclassification that occurs when individual exposure to heat is estimated using ambient temperature measured at fixed sites and can provide insights for epidemiological risk assessment concerning extreme heat.
Conclusions:
Personal heat exposure research provides more valid and precise insights into how often people encounter heat conditions and when, where, to whom, and why these encounters occur. Published literature on personal heat exposure is limited to date, but existing studies point to opportunities to inform public health practice regarding extreme heat, particularly where fine-scale precision is needed to reduce health consequences of heat exposure.