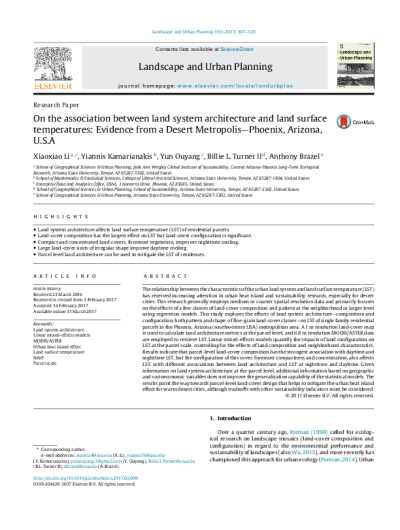
The relationship between the characteristics of the urban land system and land surface temperature (LST) has received increasing attention in urban heat island and sustainability research, especially for desert cities. This research generally employs medium or coarser spatial resolution data and primarily focuses on the effects of a few classes of land-cover composition and pattern at the neighborhood or larger level using regression models. This study explores the effects of land system architecture—composition and configuration, both pattern and shape, of fine-grain land-cover classes—on LST of single family residential parcels in the Phoenix, Arizona (southwestern USA) metropolitan area. A 1 m resolution land-cover map is used to calculate land architecture metrics at the parcel level, and 6.8 m resolution MODIS/ASTER data are employed to retrieve LST. Linear mixed-effects models quantify the impacts of land configuration on LST at the parcel scale, controlling for the effects of land composition and neighborhood characteristics. Results indicate that parcel-level land-cover composition has the strongest association with daytime and nighttime LST, but the configuration of this cover, foremost compactness and concentration, also affects LST, with different associations between land architecture and LST at nighttime and daytime. Given information on land system architecture at the parcel level, additional information based on geographic and socioeconomic variables does not improve the generalization capability of the statistical models. The results point the way towards parcel-level land-cover design that helps to mitigate the urban heat island effect for warm desert cities, although tradeoffs with other sustainability indicators must be considered.
En la zona metropolitana de Phoenix, el calor urbano está afectando la salud, la seguridad y la economía y se espera que estos impactos empeoren con el tiempo. Se prevé que el número de días por encima de 110˚F aumentará más del doble para el 2060. En mayo de 2017, The Nature Conservancy, el Departamento de Salud Pública del condado de Maricopa, Central Arizona Conservation Alliance, la Red de Investigación en Sostenibilidad sobre la Resiliencia Urbana a Eventos Extremos, el Centro de Investigación del Clima Urbano de Arizona State University y el Center for Whole Communities lanzaron un proceso participativo de planificación de acciones contra el calor para identificar tanto estrategias de mitigación como de adaptación a fin de reducir directamente el calor y mejorar la capacidad de los residentes para lidiar con el calor. Las organizaciones comunitarias con relaciones existentes en tres vecindarios seleccionados para la planificación de acciones contra el calor se unieron más tarde al equipo del proyecto: Phoenix Revitalization Corporation, RAILMesa y Puente Movement. Más allá de construir un plan de acción comunitario contra el calor y completar proyectos de demostración, este proceso participativo fue diseñado para desarrollar conciencia, iniciativa y cohesión social en las comunidades subrepresentadas. Asimismo el proceso de planificación de acciones contra el calor fue diseñado para servir como modelo para esfuerzos futuros de resiliencia al calor y crear una visión local, contextual y culturalmente apropiada de un futuro más seguro y saludable. El método iterativo de planificación y participación utilizado por el equipo del proyecto fortaleció las relaciones dentro y entre los vecindarios, las organizaciones comunitarias, los responsables de la toma de decisiones y el equipo núcleo, y combinó la sabiduría de la narración de historias y la evidencia científica para comprender mejor los desafíos actuales y futuros que enfrentan los residentes durante eventos de calor extremo. Como resultado de tres talleres en cada comunidad, los residentes presentaron ideas que quieren ver implementadas para aumentar su comodidad y seguridad térmica durante los días de calor extremo.
Como se muestra a continuación, las ideas de los residentes se interceptaron en torno a conceptos similares, pero las soluciones específicas variaron entre los vecindarios. Por ejemplo, a todos los vecindarios les gustaría agregar sombra a sus corredores peatonales, pero variaron las preferencias para la ubicación de las mejoras para dar sombra. Algunos vecindarios priorizaron las rutas de transporte público, otros priorizaron las rutas utilizadas por los niños en su camino a la escuela y otros quieren paradas de descanso con sombra en lugares clave. Surgieron cuatro temas estratégicos generales en los tres vecindarios: promover y educar; mejorar la comodidad/capacidad de afrontamiento; mejorar la seguridad; fortalecer la capacidad. Estos temas señalan que existen serios desafíos de seguridad contra el calor en la vida diaria de los residentes y que la comunidad, los negocios y los sectores responsables de la toma de decisión deben abordar esos desafíos.
Los elementos del plan de acción contra el calor están diseñados para incorporarse a otros esfuerzos para aliviar el calor, crear ciudades resilientes al clima y brindar salud y seguridad pública. Los socios de implementación del plan de acción contra el calor provienen de la región de la zona metropolitana de Phoenix, y se brindan recomendaciones para apoyar la transformación a una ciudad más fresca.
Para ampliar la escala de este enfoque, los miembros del equipo del proyecto recomiendan a) compromiso continuo e inversiones en estos vecindarios para implementar el cambio señalado como vital por los residentes, b) repetir el proceso de planificación de acción contra el calor con líderes comunitarios en otros vecindarios, y c) trabajar con las ciudades, los planificadores urbanos y otras partes interesadas para institucionalizar este proceso, apoyando las políticas y el uso de las métricas propuestas para crear comunidades más frescas.



grass) offers unique opportunities to mitigate climate change through avoided fossil fuel use and associated greenhouse gas reduction. Although conversion of existing agriculturally intensive lands (e.g., maize and soy) to perennial bioenergy cropping systems has been shown to reduce near-surface temperatures, unintended consequences on natural water resources via depletion of soil moisture may offset these benefits. In the effort of the cross-fertilization across the disciplines of physics-based modeling and spatio-temporal statistics, three topics are investigated in this dissertation aiming to provide a novel quantification and robust justifications of the hydroclimate impacts associated with bioenergy crop expansion. Topic 1 quantifies the hydroclimatic impacts associated with perennial bioenergy crop expansion over the contiguous United States using the Weather Research and Forecasting Model (WRF) dynamically coupled to a land surface model (LSM). A suite of continuous (2000–09) medium-range resolution (20-km grid spacing) ensemble-based simulations is conducted. Hovmöller and Taylor diagrams are utilized to evaluate simulated temperature and precipitation. In addition, Mann-Kendall modified trend tests and Sieve-bootstrap trend tests are performed to evaluate the statistical significance of trends in soil moisture differences. Finally, this research reveals potential hot spots of suitable deployment and regions to avoid. Topic 2 presents spatio-temporal Bayesian models which quantify the robustness of control simulation bias, as well as biofuel impacts, using three spatio-temporal correlation structures. A hierarchical model with spatially varying intercepts and slopes display satisfactory performance in capturing spatio-temporal associations. Simulated temperature impacts due to perennial bioenergy crop expansion are robust to physics parameterization schemes. Topic 3 further focuses on the accuracy and efficiency of spatial-temporal statistical modeling for large datasets. An ensemble of spatio-temporal eigenvector filtering algorithms (hereafter: STEF) is proposed to account for the spatio-temporal autocorrelation structure of the data while taking into account spatial confounding. Monte Carlo experiments are conducted. This method is then used to quantify the robustness of simulated hydroclimatic impacts associated with bioenergy crops to alternative physics parameterizations. Results are evaluated against those obtained from three alternative Bayesian spatio-temporal specifications.


The tool allowed surgeons to take an allograft reconstruction and fuse it to a patient’s CT or MR medical image for virtual fit assessment. The allograft is either a reconstruction of the donor’s actual heart (from CT or MR images) or an analogue from a health heart library. The analogue allograft geometry is identified from gross donor parameters using a regression model build herein. The need for the regression model is donor images may not exist or they may not become available within the time-window clinicians have to make a provisional acceptance of an offer.
The tool’s assessment suggested > 20% of upper DRBW listings could have been increased at Phoenix Children’s Hospital (PCH). Upper DRBW listings in the UNOS national database was statistically smaller than at PCH (p-values: < 0.001). Delayed sternal closure and surgeon perceived complication variables had an association (p-value: 0.000016) with 9 of the 11 cases that surgeons had perceived fit-related complications had delayed closures (p-value: 0.034809).
A tool to assess allograft size-match has been developed. Findings warrant future preclinical and clinical prospective studies to further assess the tool’s clinical utility.
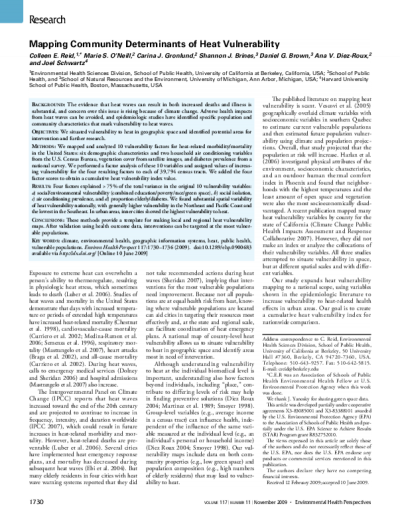
Background:
The evidence that heat waves can result in both increased deaths and illness is substantial, and concern over this issue is rising because of climate change. Adverse health impacts from heat waves can be avoided, and epidemiologic studies have identified specific population and community characteristics that mark vulnerability to heat waves.
Objectives:
We situated vulnerability to heat in geographic space and identified potential areas for intervention and further research.
Methods:
We mapped and analyzed 10 vulnerability factors for heat-related morbidity/mortality in the United States: six demographic characteristics and two household air conditioning variables from the U.S. Census Bureau, vegetation cover from satellite images, and diabetes prevalence from a national survey. We performed a factor analysis of these 10 variables and assigned values of increasing vulnerability for the four resulting factors to each of 39,794 census tracts. We added the four factor scores to obtain a cumulative heat vulnerability index value.
Results:
Four factors explained > 75% of the total variance in the original 10 vulnerability variables: a) social/environmental vulnerability (combined education/poverty/race/green space), b) social isolation, c) air conditioning prevalence, and d) proportion elderly/diabetes. We found substantial spatial variability of heat vulnerability nationally, with generally higher vulnerability in the Northeast and Pacific Coast and the lowest in the Southeast. In urban areas, inner cities showed the highest vulnerability to heat.
Conclusions:
These methods provide a template for making local and regional heat vulnerability maps. After validation using health outcome data, interventions can be targeted at the most vulnerable populations.
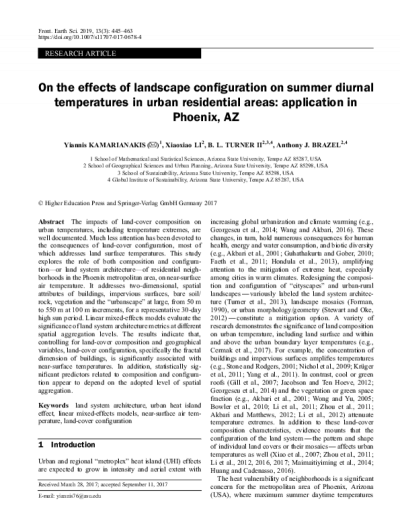
The impacts of land-cover composition on urban temperatures, including temperature extremes, are well documented. Much less attention has been devoted to the consequences of land-cover configuration, most of which addresses land surface temperatures. This study explores the role of both composition and configuration—or land system architecture—of residential neighborhoods in the Phoenix metropolitan area, on near-surface air temperature. It addresses two-dimensional, spatial attributes of buildings, impervious surfaces, bare soil/rock, vegetation and the “urbanscape” at large, from 50 m to 550 m at 100 m increments, for a representative 30-day high sun period. Linear mixed-effects models evaluate the significance of land system architecture metrics at different spatial aggregation levels. The results indicate that, controlling for land-cover composition and geographical variables, land-cover configuration, specifically the fractal dimension of buildings, is significantly associated with near-surface temperatures. In addition, statistically significant predictors related to composition and configuration appear to depend on the adopted level of spatial aggregation.