Matching Items (159)

Description
MicroRNAs (miRNAs) are 17-22 nucleotide non-coding RNAs that regulate gene expression by targeting non-complementary elements in the 3’ untranslated regions (3’UTRs) of mRNAs. miRNAs, which form complex networks of interaction that differ by tissue and developmental stage, display conservation in their function across metazoan species. Yet much remains unknown regarding their biogenesis, localization, strand selection, and their absolute abundance due to the difficulty of detecting and amplifying such small molecules. Here, I used an updated HT qPCR-based methodology to follow miRNA expression of 5p and 3p strands for all 190 C. elegans miRNAs described in miRBase throughout all six developmental stages in triplicates (total of 9,708 experiments), and studied their expression levels, tissue localization, and the rules underlying miRNA strand selection. My study validated previous findings and identified novel, conserved patterns of miRNA strand expression throughout C. elegans development, which at times correlate with previously observed developmental phenotypes. Additionally, my results highlighted novel structural principles underlying strand selection, which can be applied to higher metazoans.
Though optimized for use in C. elegans, this method can be easily adapted to other eukaryotic systems, allowing for more scalable quantitative investigation of miRNA biology and/or miRNA diagnostics.
ContributorsMeadows, Dalton Alexander (Author) / Mangone, Marco (Thesis advisor) / LaBaer, Joshua (Committee member) / Murugan, Vel (Committee member) / Wilson-Rawls, Jeanne (Committee member) / Arizona State University (Publisher)
Created2023
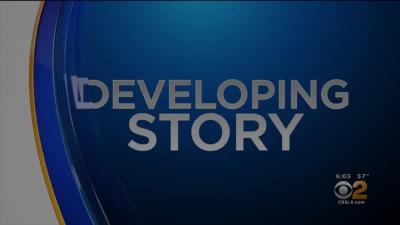
Description
Under the direction of Dr. Carolyn Compton, a group of seven Barrett honors students have embarked on a truly unique team thesis project to create a documentary on the process of creating a COVID-19 testing laboratory. This documentary tells the story of the ASU Biodesign Clinical Testing Laboratory (ABCTL), the first lab in the western United States to offer public saliva testing to identify the presence of COVID-19.
ContributorsCura, Joriel (Director, Photographer) / Foote, Hannah (Producer, Sound designer) / Raymond, Julia (Production personnel) / Bardfeld, Sierra (Narrator, Editor) / Dholaria, Nikhil (Writer of added commentary) / Liu, Tara (Writer of added commentary) / Varghese, Mahima (Writer of added commentary) / Compton, Carolyn C. (Interviewee, Project director) / Harris, Valerie (Interviewee) / LaBaer, Joshua (Interviewee) / Miceli, Joseph (Interviewee) / Nelson, Megan (Interviewee) / Ungaro, Brianna (Interviewee)
Created2021

Description
The purpose of the overall project is to create a simulated environment similar to Google map and traffic but simplified for education purposes. Students can choose different traffic patterns and program a car to navigate through the traffic dynamically based on the changing traffic. The environment used in the project is ASU VIPLE (Visual IoT/Robotics Programming Language Environment). It is a visual programming environment for Computer Science education. VIPLE supports a number of devices and platforms, including a traffic simulator developed using Unity game engine. This thesis focuses on creating realistic traffic data for the traffic simulator and implementing dynamic routing algorithm in VIPLE. The traffic data is generated from the recorded real traffic data published at Arizona Maricopa County website. Based on the generated traffic data, VIPLE programs are developed to implement the traffic simulation based on dynamic changing traffic data.
ContributorsZhang, Zhemin (Author) / Chen, Yinong (Thesis advisor) / Wang, Yalin (Thesis advisor) / De Luca, Gennaro (Committee member) / Arizona State University (Publisher)
Created2022

Description
Little is known about how cognitive and brain aging patterns differ in older adults with autism spectrum disorder (ASD). However, recent evidence suggests that individuals with ASD may be at greater risk of pathological aging conditions than their neurotypical (NT) counterparts. A growing body of research indicates that older adults with ASD may experience accelerated cognitive decline and neurodegeneration as they age, although studies are limited by their cross-sectional design in a population with strong age-cohort effects. Studying aging in ASD and identifying biomarkers to predict atypical aging is important because the population of older individuals with ASD is growing. Understanding the unique challenges faced as autistic adults age is necessary to develop treatments to improve quality of life and preserve independence. In this study, a longitudinal design was used to characterize cognitive and brain aging trajectories in ASD as a function of autistic trait severity. Principal components analysis (PCA) was used to derive a cognitive metric that best explains performance variability on tasks measuring memory ability and executive function. The slope of the integrated persistent feature (SIP) was used to quantify functional connectivity; the SIP is a novel, threshold-free graph theory metric which summarizes the speed of information diffusion in the brain. Longitudinal mixed models were using to predict cognitive and brain aging trajectories (measured via the SIP) as a function of autistic trait severity, sex, and their interaction. The sensitivity of the SIP was also compared with traditional graph theory metrics. It was hypothesized that older adults with ASD would experience accelerated cognitive and brain aging and furthermore, age-related changes in brain network topology would predict age-related changes in cognitive performance.
For both cognitive and brain aging, autistic traits and sex interacted to predict trajectories, such that older men with high autistic traits were most at risk for poorer outcomes. In men with autism, variability in SIP scores across time points trended toward predicting cognitive aging trajectories. Findings also suggested that autistic traits are more sensitive to differences in brain aging than diagnostic group and that the SIP is more sensitive to brain aging trajectories than other graph theory metrics. However, further research is required to determine how physiological biomarkers such as the SIP are associated with cognitive outcomes.
ContributorsSullivan, Georgia (Author) / Braden, Blair (Thesis advisor) / Kodibagkar, Vikram (Thesis advisor) / Schaefer, Sydney (Committee member) / Wang, Yalin (Committee member) / Arizona State University (Publisher)
Created2022

Description
As robots become increasingly integrated into the environments, they need to learn how to interact with the objects around them. Many of these objects are articulated with multiple degrees of freedom (DoF). Multi-DoF objects have complex joints that require specific manipulation orders, but existing methods only consider objects with a single joint. To capture the joint structure and manipulation sequence of any object, I introduce the "Object Kinematic State Machines" (OKSMs), a novel representation that models the kinematic constraints and manipulation sequences of multi-DoF objects. I also present Pokenet, a deep neural network architecture that estimates the OKSMs from the sequence of point cloud data of human demonstrations. I conduct experiments on both simulated and real-world datasets to validate my approach. First, I evaluate the modeling of multi-DoF objects on a simulated dataset, comparing against the current state-of-the-art method. I then assess Pokenet's real-world usability on a dataset collected in my lab, comprising 5,500 data points across 4 objects. Results showcase that my method can successfully estimate joint parameters of novel multi-DoF objects with over 25% more accuracy on average than prior methods.
ContributorsGUPTA, ANMOL (Author) / Gopalan, Nakul (Thesis advisor) / Zhang, Yu (Committee member) / Wang, Yalin (Committee member) / Arizona State University (Publisher)
Created2024

Description
In today's data-driven world, privacy is a significant concern. It is crucial to preserve the privacy of sensitive information while visualizing data. This thesis aims to develop new techniques and software tools that support Vega-Lite visualizations while maintaining privacy. Vega-Lite is a visualization grammar based on Wilkinson's grammar of graphics. The project extends Vega-Lite to incorporate privacy algorithms such as k-anonymity, l-diversity, t-closeness, and differential privacy. This is done by using a unique multi-input loop module logic that generates combinations of attributes as a new anonymization method. Differential privacy is implemented by adding controlled noise (Laplace or Exponential) to the sensitive columns in the dataset. The user defines custom rules in the JSON schema, mentioning the privacy methods and the sensitive column. The schema is validated using Another JSON Validation library, and these rules help identify the anonymization techniques to be performed on the dataset before sending it back to the Vega-Lite visualization server. Multiple datasets satisfying the privacy requirements are generated, and their utility scores are provided so that the user can trade-off between privacy and utility on the datasets based on their requirements. The interface developed is user-friendly and intuitive and guides users in using it. It provides appropriate feedback on the privacy-preserving visualizations generated through various utility metrics. This application is helpful for technical or domain experts across multiple domains where privacy is a big concern, such as medical institutions, traffic and urban planning, financial institutions, educational records, and employer-employee relations. This project is novel as it provides a one-stop solution for privacy-preserving visualization. It works on open-source software, Vega-Lite, which several organizations and users use for business and educational purposes.
ContributorsSekar, Manimozhi (Author) / Bryan, Chris (Thesis advisor) / Wang, Yalin (Committee member) / Cao, Zhichao (Committee member) / Arizona State University (Publisher)
Created2024

Description
Image denoising, a fundamental task in computer vision, poses significant challenges due to its inherently inverse and ill-posed nature. Despite advancements in traditional methods and supervised learning approaches, particularly in medical imaging such as Medical Resonance Imaging (MRI) scans, the reliance on paired datasets and known noise distributions remains a practical hurdle. Recent progress in noise statistical independence theory and diffusion models has revitalized research interest, offering promising avenues for unsupervised denoising. However, existing methods often yield overly smoothed results or introduce hallucinated structures, limiting their clinical applicability. This thesis tackles the core challenge of progressing towards unsupervised denoising of MRI scans. It aims to retain intricate details without smoothing or introducing artificial structures, thus ensuring the production of high-quality MRI images. The thesis makes a three-fold contribution: Firstly, it presents a detailed analysis of traditional techniques, early machine learning algorithms for denoising, and new statistical-based models, with an extensive evaluation study on self-supervised denoising methods highlighting their limitations. Secondly, it conducts an evaluation study on an emerging class of diffusion-based denoising methods, accompanied by additional empirical findings and discussions on their effectiveness and limitations, proposing solutions to enhance their utility. Lastly, it introduces a novel approach, Unsupervised Multi-stage Ensemble Deep Learning with diffusion models for denoising MRI scans (MEDL). Leveraging diffusion models, this approach operates independently of signal or noise priors and incorporates weighted rescaling of multi-stage reconstructions to balance over-smoothing and hallucination tendencies. Evaluation using benchmark datasets demonstrates an average gain of 1dB and 2% in PSNR and SSIM metrics, respectively, over existing approaches.
ContributorsVora, Sahil (Author) / Li, Baoxin (Thesis advisor) / Wang, Yalin (Committee member) / Zhou, Yuxiang (Committee member) / Arizona State University (Publisher)
Created2024

Description
Unsupervised learning of time series data, also known as temporal clustering, is a challenging problem in machine learning. This thesis presents a novel algorithm, Deep Temporal Clustering (DTC), to naturally integrate dimensionality reduction and temporal clustering into a single end-to-end learning framework, fully unsupervised. The algorithm utilizes an autoencoder for temporal dimensionality reduction and a novel temporal clustering layer for cluster assignment. Then it jointly optimizes the clustering objective and the dimensionality reduction objective. Based on requirement and application, the temporal clustering layer can be customized with any temporal similarity metric. Several similarity metrics and state-of-the-art algorithms are considered and compared. To gain insight into temporal features that the network has learned for its clustering, a visualization method is applied that generates a region of interest heatmap for the time series. The viability of the algorithm is demonstrated using time series data from diverse domains, ranging from earthquakes to spacecraft sensor data. In each case, the proposed algorithm outperforms traditional methods. The superior performance is attributed to the fully integrated temporal dimensionality reduction and clustering criterion.
ContributorsMadiraju, NaveenSai (Author) / Liang, Jianming (Thesis advisor) / Wang, Yalin (Thesis advisor) / He, Jingrui (Committee member) / Arizona State University (Publisher)
Created2018

Description
Signal transduction networks comprising protein-protein interactions (PPIs) mediate homeostatic, diseased, and therapeutic cellular responses. Mapping these networks has primarily focused on identifying interactors, but less is known about the interaction affinity, rates of interaction or their regulation. To better understand the extent of the annotated human interactome, I first examined > 2500 protein interactions within the B cell receptor (BCR) signaling pathway using a current, cutting-edge bioluminescence-based platform called “NanoBRET” that is capable of analyzing transient and stable interactions in high throughput. Eighty-three percent (83%) of the detected interactions have not been previously reported, indicating that much of the BCR pathway is still unexplored. Unfortunately, NanoBRET, as with all other high throughput methods, cannot determine binding kinetics or affinities. To address this shortcoming, I developed a hybrid platform that characterizes > 400 PPIs quantitatively and simultaneously in < 1 hour by combining the high throughput and flexible nature of nucleic programmable protein arrays (NAPPA) with the quantitative abilities of surface plasmon resonance imaging (SPRi). NAPPA-SPRi was then used to study the kinetics and affinities of > 12,000 PPIs in the BCR signaling pathway, revealing unique kinetic mechanisms that are employed by proteins, phosphorylation and activation states to regulate PPIs. In one example, activation of the GTPase RAC1 with nonhydrolyzable GTP-γS minimally affected its binding affinities with phosphorylated proteins but increased, on average, its on- and off-rates by 4 orders of magnitude for one-third of its interactions. In contrast, this phenomenon occurred with virtually all unphosphorylated proteins. The majority of the interactions (85%) were novel, sharing 40% of the same interactions as NanoBRET as well as detecting 55% more interactions than NanoBRET. In addition, I further validated four novel interactions identified by NAPPA-SPRi using SDS-PAGE migration and Western blot analyses. In one case, we have the first evidence of a direct enzyme-substrate interaction between two well-known proto-oncogenes that are abnormally regulated in > 30% of cancers, PI3K and MYC. Herein, PI3K is demonstrated to phosphorylate MYC at serine 62, a phosphosite that increases the stability of MYC. This study provides valuable insight into how PPIs, phosphorylation, and GTPase activation regulate the BCR signal transduction pathway. In addition, these methods could be applied toward understanding other signaling pathways, pathogen-host interactions, and the effect of protein mutations on protein interactions.
ContributorsPetritis, Brianne Ogata (Author) / LaBaer, Joshua (Thesis advisor) / Lake, Douglas (Committee member) / Wang, Shaopeng (Committee member) / Arizona State University (Publisher)
Created2018
Description
According to the World Health Organization, cancer is one of the leading causes of death around the world. Although early diagnostics using biomarkers and improved treatments with targeted therapy have reduced the rate of cancer related mortalities, there remain many unknowns regarding the contributions of the tumor microenvironment to cancer progression and therapeutic resistance. The tumor microenvironment plays a significant role by manipulating the progression of cancer cells through biochemical and biophysical signals from the surrounding stromal cells along with the extracellular matrix. As such, there is a critical need to understand how the tumor microenvironment influences the molecular mechanisms underlying cancer metastasis to facilitate the discovery of better therapies. This thesis described the development of microfluidic technologies to study the interplay of cancer cells with their surrounding microenvironment. The microfluidic model was used to assess how exposure to chemoattractant, epidermal growth factor (EGF), impacted 3D breast cancer cell invasion and enhanced cell motility speed was noted in the presence of EGF validating physiological cell behavior. Additionally, breast cancer and patient-derived cancer-associated fibroblast (CAF) cells were co-cultured to study cell-cell crosstalk and how it affected cancer invasion. GPNMB was identified as a novel gene of interest and it was shown that CAFs enhanced breast cancer invasion by up-regulating the expression of GPNMB on breast cancer cells resulting in increased migration speed. Lastly, this thesis described the design, biological validation, and use of this microfluidic platform as a new in vitro 3D organotypic model to study mechanisms of glioma stem cell (GSC) invasion in the context of a vascular niche. It was confirmed that CXCL12-CXCR4 signaling is involved in promoting GSC invasion in a 3D vascular microenvironment, while also demonstrating the effectiveness of the microfluidic as a drug screening assay. Taken together, the broader impacts of the microfluidic model developed in this dissertation include, a possible alternative platform to animal testing that is focused on mimicking human physiology, a potential ex vivo platform using patient-derived cells for studying the interplay of cancer cells with its surrounding microenvironment, and development of future therapeutic strategies tailored toward disrupting key molecular pathways involved in regulatory mechanisms of cancer invasion.
ContributorsTruong, Danh, Ph.D (Author) / Nikkhah, Mehdi (Thesis advisor) / LaBaer, Joshua (Committee member) / Smith, Barbara (Committee member) / Mouneimne, Ghassan (Committee member) / Vernon, Brent (Committee member) / Arizona State University (Publisher)
Created2018