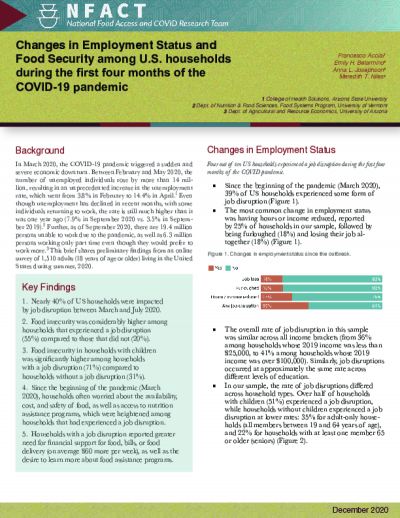
In March 2020, the COVID-19 pandemic triggered a sudden and severe economic downturn. Between February and May 2020, the number of unemployed individuals rose by more than 14 million, resulting in an unprecedented increase in the unemployment rate, which went from 3.8% in February to 14.4% in April. Even though unemployment has declined in recent months, with some individuals returning to work, the rate is still much higher than it was one year ago (7.9% in September 2020 vs. 3.5% in September 2019). Further, as of September 2020, there are 19.4 million persons unable to work due to the pandemic, as well as 6.3 million persons working only part time even though they would prefer to work more.
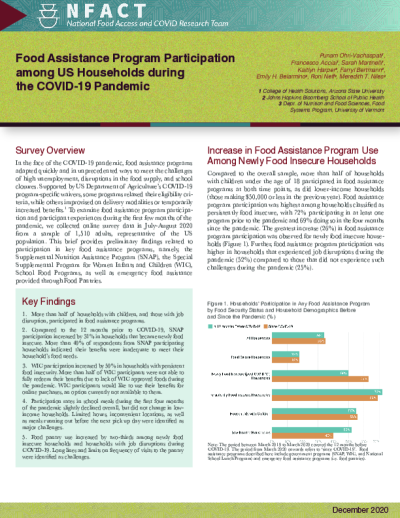
In the face of the coronavirus (COVID-19) pandemic, food assistance programs adapted quickly and in unprecedented ways to meet the challenges of high unemployment, disruptions in the food supply, and school closures. Supported by US Department of Agriculture’s COVID-19 program-specific waivers, some programs relaxed their eligibility criteria, while others improvised on delivery modalities or temporarily increased benefits.1 To examine food assistance program participation and participant experiences during the first few months of the pandemic, we collected online survey data in July 2020 from a sample of over 1,500 U.S. households, representative of the US population. This brief summarizes participation in key food assistance programs, namely, the Supplemental Nutrition Assistance Program (SNAP), the Special Supplemental Program for Women Infants and Children (WIC), School Food Programs, as well as emergency food assistance provided through Food Pantries.
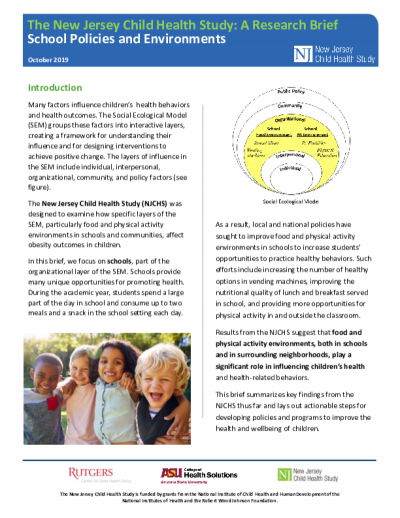
Many factors influence children’s health behaviors and health outcomes. The Social Ecological Model (SEM) groups these factors into interactive layers, creating a framework for understanding their influence and for designing interventions to achieve positive change. The layers of influence in the SEM include individual, interpersonal, organizational, community, and policy factors.
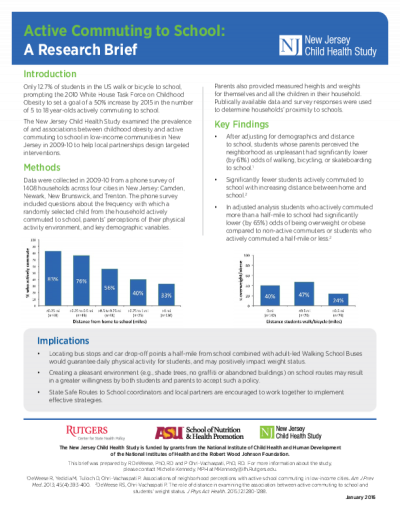
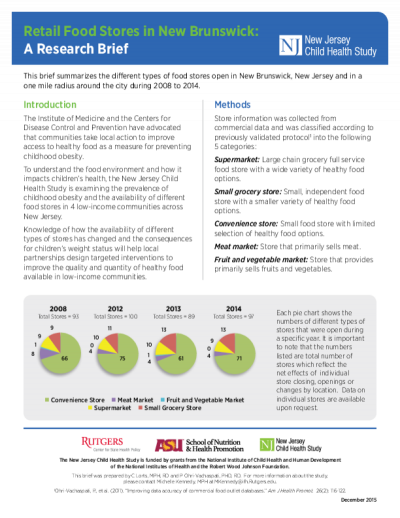
This brief summarizes the different types of food stores open in New Brunswick, New Jersey and in a one mile radius around the city during 2008 to 2014.
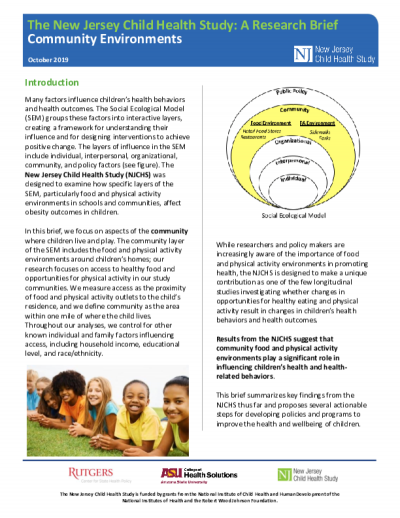
Many factors influence children’s health behaviors and health outcomes. The Social Ecological Model (SEM) groups these factors into interactive layers, creating a framework for understanding their influence and for designing interventions to achieve positive change. The layers of influence in the SEM include individual, interpersonal, organizational, community, and policy factors (see figure). The New Jersey Child Health Study (NJCHS) was designed to examine how specific layers of the SEM, particularly food and physical activity environments in schools and communities, affect obesity outcomes in children
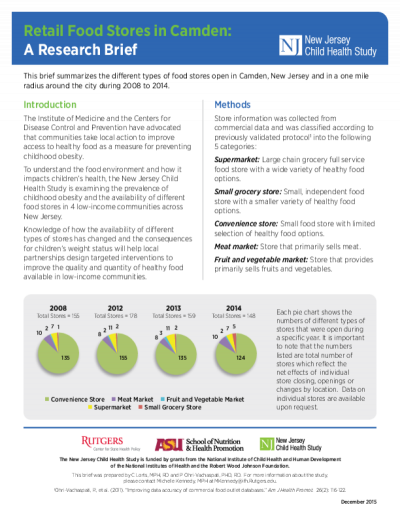
This brief summarizes the different types of food stores open in Camden, New Jersey and in a one mile radius around the city during 2008 to 2014.
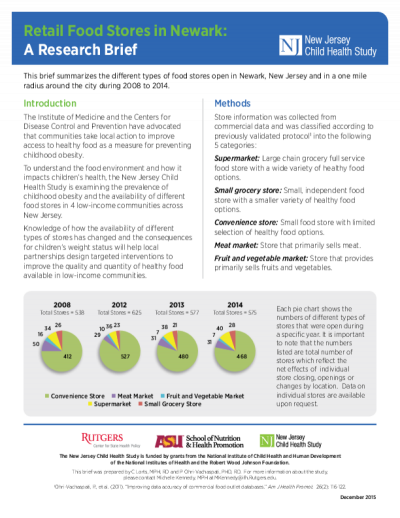
This brief summarizes the different types of food stores open in Newark, New Jersey and in a one mile radius around the city during 2008 to 2014.
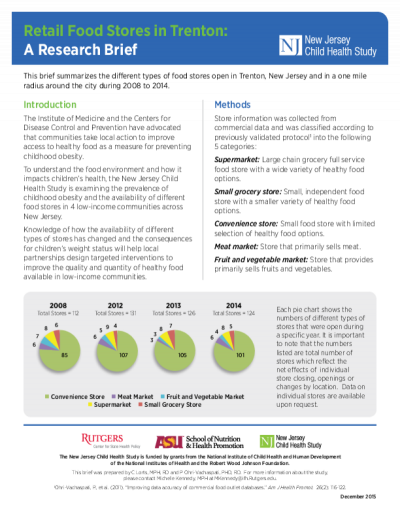
This brief summarizes the different types of food stores open in Trenton, New Jersey and in a one mile radius around the city during 2008 to 2014.

Aims: to evaluate 1) the PA variation explained by work walkability, 2) the moderating effects of person-level characteristics to the relationship between PA and work walkability, and 3) the differences in the rate of change in PA over time by worksite walkability.
Methods: self-report and accelerometer measured PA at baseline (aim 1, 2); longitudinal accelerometer PA during the initial 56 days of a behavioral intervention (aim 3). Adults were generally healthy and reported part- or full-time employment with a geocodeable address outside the home. Geographic Information Systems (GIS) measured walkability followed established techniques (i.e., residential, intersection, and transit densities, and land-use-mix).
Results: On average, worksite walkability did not show direct relationships with PA (aim 1); yet certain person-level characteristics moderated the relationships: sex, race, and not having young children in the household (aim 2). During 56 days of intervention, the PA rate of change over time showed no evidence of a moderating effect by worksite walkability.
Discussion: Worksite walkability was generally not shown to relate to the overall PA. However, specific subgroups (women, those without young children) appeared more responsive to their worksite neighborhood walkability. Prior literature shows certain demographics respond differently with various BE exposures, and this study adds a potentially novel moderator of interest regarding young children at home. Understanding who benefits from access to walkable BE may inform targeted interventions and policy to improve PA levels and foster health equity.