Filtering by
- Language: English





En la zona metropolitana de Phoenix, el calor urbano está afectando la salud, la seguridad y la economía y se espera que estos impactos empeoren con el tiempo. Se prevé que el número de días por encima de 110˚F aumentará más del doble para el 2060. En mayo de 2017, The Nature Conservancy, el Departamento de Salud Pública del condado de Maricopa, Central Arizona Conservation Alliance, la Red de Investigación en Sostenibilidad sobre la Resiliencia Urbana a Eventos Extremos, el Centro de Investigación del Clima Urbano de Arizona State University y el Center for Whole Communities lanzaron un proceso participativo de planificación de acciones contra el calor para identificar tanto estrategias de mitigación como de adaptación a fin de reducir directamente el calor y mejorar la capacidad de los residentes para lidiar con el calor. Las organizaciones comunitarias con relaciones existentes en tres vecindarios seleccionados para la planificación de acciones contra el calor se unieron más tarde al equipo del proyecto: Phoenix Revitalization Corporation, RAILMesa y Puente Movement. Más allá de construir un plan de acción comunitario contra el calor y completar proyectos de demostración, este proceso participativo fue diseñado para desarrollar conciencia, iniciativa y cohesión social en las comunidades subrepresentadas. Asimismo el proceso de planificación de acciones contra el calor fue diseñado para servir como modelo para esfuerzos futuros de resiliencia al calor y crear una visión local, contextual y culturalmente apropiada de un futuro más seguro y saludable. El método iterativo de planificación y participación utilizado por el equipo del proyecto fortaleció las relaciones dentro y entre los vecindarios, las organizaciones comunitarias, los responsables de la toma de decisiones y el equipo núcleo, y combinó la sabiduría de la narración de historias y la evidencia científica para comprender mejor los desafíos actuales y futuros que enfrentan los residentes durante eventos de calor extremo. Como resultado de tres talleres en cada comunidad, los residentes presentaron ideas que quieren ver implementadas para aumentar su comodidad y seguridad térmica durante los días de calor extremo.
Como se muestra a continuación, las ideas de los residentes se interceptaron en torno a conceptos similares, pero las soluciones específicas variaron entre los vecindarios. Por ejemplo, a todos los vecindarios les gustaría agregar sombra a sus corredores peatonales, pero variaron las preferencias para la ubicación de las mejoras para dar sombra. Algunos vecindarios priorizaron las rutas de transporte público, otros priorizaron las rutas utilizadas por los niños en su camino a la escuela y otros quieren paradas de descanso con sombra en lugares clave. Surgieron cuatro temas estratégicos generales en los tres vecindarios: promover y educar; mejorar la comodidad/capacidad de afrontamiento; mejorar la seguridad; fortalecer la capacidad. Estos temas señalan que existen serios desafíos de seguridad contra el calor en la vida diaria de los residentes y que la comunidad, los negocios y los sectores responsables de la toma de decisión deben abordar esos desafíos.
Los elementos del plan de acción contra el calor están diseñados para incorporarse a otros esfuerzos para aliviar el calor, crear ciudades resilientes al clima y brindar salud y seguridad pública. Los socios de implementación del plan de acción contra el calor provienen de la región de la zona metropolitana de Phoenix, y se brindan recomendaciones para apoyar la transformación a una ciudad más fresca.
Para ampliar la escala de este enfoque, los miembros del equipo del proyecto recomiendan a) compromiso continuo e inversiones en estos vecindarios para implementar el cambio señalado como vital por los residentes, b) repetir el proceso de planificación de acción contra el calor con líderes comunitarios en otros vecindarios, y c) trabajar con las ciudades, los planificadores urbanos y otras partes interesadas para institucionalizar este proceso, apoyando las políticas y el uso de las métricas propuestas para crear comunidades más frescas.
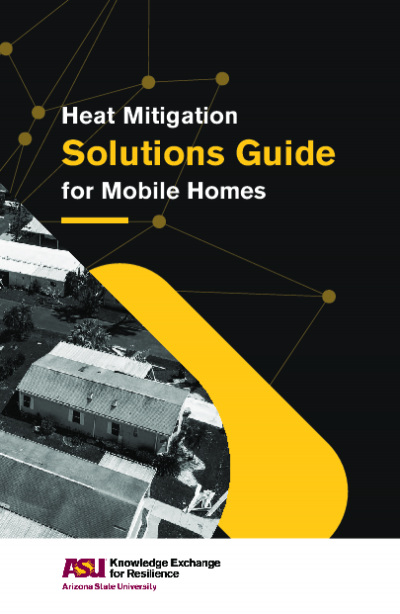
According to the Centers for Disease Control and Prevention (CDC), more people die in the U.S. from heat than from all other natural disasters combined. According to the Environmental Protection Agency (EPA), more than 1,300 deaths per year in the United States are due to extreme heat. Arizona, California and Texas are the three states with the highest burden, accounting for 43% of all heat-related deaths according to the CDC.
Although only 5% of housing in Maricopa County, Arizona, is mobile homes, approximately 30% of indoor heat-related deaths occur in these homes. Thus, the residents of mobile homes in Maricopa County are disproportionately affected by heat. Mobile home residents are extremely exposed to heat due to the high density of mobile home parks, poor construction of dwellings, lack of vegetation, socio-demographic features and not being eligible to get utility and financial assistance.
We researched numerous solutions across different domains that could help build the heat resilience of mobile home residents. As a result we found 50 different solutions for diverse stakeholders, budgets and available resources. The goal of this toolbox is to present these solutions and to explain how to apply them in order to get the most optimal result and build About this Solutions Guide People who live in mobile homes are 6 to 8 times more likely to die of heat-associated deaths. heat resilience for mobile home residents. These solutions were designed as a coordinated set of actions for everyone — individual households, mobile home residents, mobile home park owners, cities and counties, private businesses and nonprofits serving mobile home parks, and other stakeholders — to be able to contribute to heat mitigation for mobile home residents.
When we invest in a collective, coordinated suite of solutions that are designed specifically to address the heat vulnerability of mobile homes residents, we can realize a resilience dividend in maintaining affordable, feasible, liveable housing for the 20 million Americans who choose mobile homes and manufactured housing as their place to live and thrive.

