Filtering by
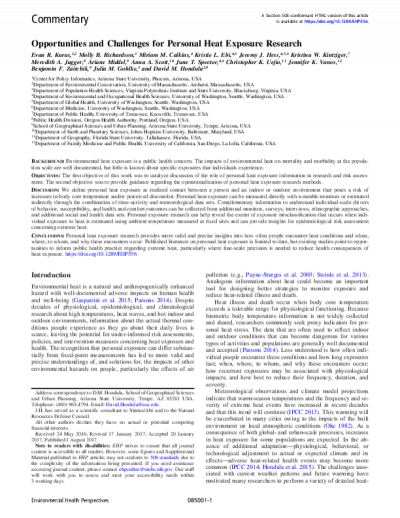
Background:
Environmental heat exposure is a public health concern. The impacts of environmental heat on mortality and morbidity at the population scale are well documented, but little is known about specific exposures that individuals experience.
Objectives:
The first objective of this work was to catalyze discussion of the role of personal heat exposure information in research and risk assessment. The second objective was to provide guidance regarding the operationalization of personal heat exposure research methods.
Discussion:
We define personal heat exposure as realized contact between a person and an indoor or outdoor environment that poses a risk of increases in body core temperature and/or perceived discomfort. Personal heat exposure can be measured directly with wearable monitors or estimated indirectly through the combination of time–activity and meteorological data sets. Complementary information to understand individual-scale drivers of behavior, susceptibility, and health and comfort outcomes can be collected from additional monitors, surveys, interviews, ethnographic approaches, and additional social and health data sets. Personal exposure research can help reveal the extent of exposure misclassification that occurs when individual exposure to heat is estimated using ambient temperature measured at fixed sites and can provide insights for epidemiological risk assessment concerning extreme heat.
Conclusions:
Personal heat exposure research provides more valid and precise insights into how often people encounter heat conditions and when, where, to whom, and why these encounters occur. Published literature on personal heat exposure is limited to date, but existing studies point to opportunities to inform public health practice regarding extreme heat, particularly where fine-scale precision is needed to reduce health consequences of heat exposure.

Background:
Data assimilation refers to methods for updating the state vector (initial condition) of a complex spatiotemporal model (such as a numerical weather model) by combining new observations with one or more prior forecasts. We consider the potential feasibility of this approach for making short-term (60-day) forecasts of the growth and spread of a malignant brain cancer (glioblastoma multiforme) in individual patient cases, where the observations are synthetic magnetic resonance images of a hypothetical tumor.
Results:
We apply a modern state estimation algorithm (the Local Ensemble Transform Kalman Filter), previously developed for numerical weather prediction, to two different mathematical models of glioblastoma, taking into account likely errors in model parameters and measurement uncertainties in magnetic resonance imaging. The filter can accurately shadow the growth of a representative synthetic tumor for 360 days (six 60-day forecast/update cycles) in the presence of a moderate degree of systematic model error and measurement noise.
Conclusions:
The mathematical methodology described here may prove useful for other modeling efforts in biology and oncology. An accurate forecast system for glioblastoma may prove useful in clinical settings for treatment planning and patient counseling.
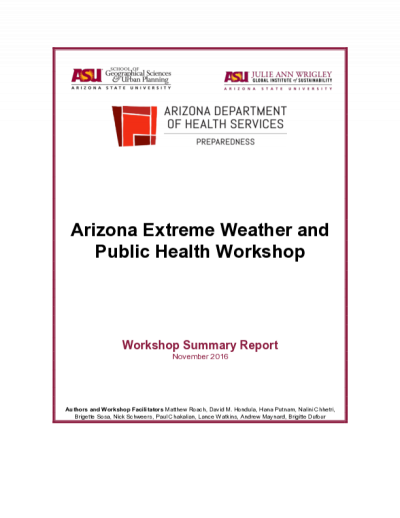
This report summarizes the proceedings of the workshop focusing primarily on two sessions: the first related to social vulnerability mapping and the second related to the identification and prioritization of interventions necessary to address the impacts of climate-sensitive hazards.
