Filtering by
- All Subjects: Sentiment Analysis
- Creators: Bansal, Ajay
- Creators: Bellis, Camellia

To facilitate rapid, correct, efficient, and intuitive development of graph based solutions we propose a new programming language construct - the search statement. Given a supra-root node, a procedure which determines the children of a given parent node, and optional definitions of the fail-fast acceptance or rejection of a solution, the search statement can conduct a search over any graph or network. Structurally, this statement is modelled after the common switch statement and is put into a largely imperative/procedural context to allow for immediate and intuitive development by most programmers. The Go programming language has been used as a foundation and proof-of-concept of the search statement. A Go compiler is provided which implements this construct.

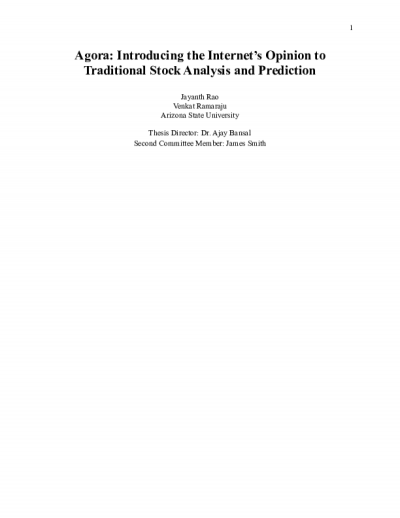
This project aims to incorporate the aspect of sentiment analysis into traditional stock analysis to enhance stock rating predictions by applying a reliance on the opinion of various stocks from the Internet. Headlines from eight major news publications and conversations from Yahoo! Finance’s “Conversations” feature were parsed through the Valence Aware Dictionary for Sentiment Reasoning (VADER) natural language processing package to determine numerical polarities which represented positivity or negativity for a given stock ticker. These generated polarities were paired with stock metrics typically observed by stock analysts as the feature set for a Logistic Regression machine learning model. The model was trained on roughly 1500 major stocks to determine a binary classification between a “Buy” or “Not Buy” rating for each stock, and the results of the model were inserted into the back-end of the Agora Web UI which emulates search engine behavior specifically for stocks found in NYSE and NASDAQ. The model reported an accuracy of 82.5% and for most major stocks, the model’s prediction correlated with stock analysts’ ratings. Given the volatility of the stock market and the propensity for hive-mind behavior in online forums, the performance of the Logistic Regression model would benefit from incorporating historical stock data and more sources of opinion to balance any subjectivity in the model.

This project aims to incorporate the aspect of sentiment analysis into traditional stock analysis to enhance stock rating predictions by applying a reliance on the opinion of various stocks from the Internet. Headlines from eight major news publications and conversations from Yahoo! Finance’s “Conversations” feature were parsed through the Valence Aware Dictionary for Sentiment Reasoning (VADER) natural language processing package to determine numerical polarities which represented positivity or negativity for a given stock ticker. These generated polarities were paired with stock metrics typically observed by stock analysts as the feature set for a Logistic Regression machine learning model. The model was trained on roughly 1500 major stocks to determine a binary classification between a “Buy” or “Not Buy” rating for each stock, and the results of the model were inserted into the back-end of the Agora Web UI which emulates search engine behavior specifically for stocks found in NYSE and NASDAQ. The model reported an accuracy of 82.5% and for most major stocks, the model’s prediction correlated with stock analysts’ ratings. Given the volatility of the stock market and the propensity for hive-mind behavior in online forums, the performance of the Logistic Regression model would benefit from incorporating historical stock data and more sources of opinion to balance any subjectivity in the model.