Filtering by
- All Subjects: Machine Learning
- Creators: Computer Science and Engineering Program
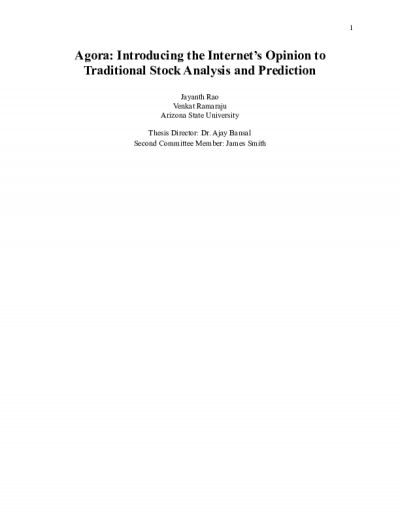
This project aims to incorporate the aspect of sentiment analysis into traditional stock analysis to enhance stock rating predictions by applying a reliance on the opinion of various stocks from the Internet. Headlines from eight major news publications and conversations from Yahoo! Finance’s “Conversations” feature were parsed through the Valence Aware Dictionary for Sentiment Reasoning (VADER) natural language processing package to determine numerical polarities which represented positivity or negativity for a given stock ticker. These generated polarities were paired with stock metrics typically observed by stock analysts as the feature set for a Logistic Regression machine learning model. The model was trained on roughly 1500 major stocks to determine a binary classification between a “Buy” or “Not Buy” rating for each stock, and the results of the model were inserted into the back-end of the Agora Web UI which emulates search engine behavior specifically for stocks found in NYSE and NASDAQ. The model reported an accuracy of 82.5% and for most major stocks, the model’s prediction correlated with stock analysts’ ratings. Given the volatility of the stock market and the propensity for hive-mind behavior in online forums, the performance of the Logistic Regression model would benefit from incorporating historical stock data and more sources of opinion to balance any subjectivity in the model.
Standardization is sorely lacking in the field of musical machine learning. This thesis project endeavors to contribute to this standardization by training three machine learning models on the same dataset and comparing them using the same metrics. The music-specific metrics utilized provide more relevant information for diagnosing the shortcomings of each model.



User interface development on iOS is in a major transitionary state as Apple introduces a declarative and interactive framework called SwiftUI. SwiftUI’s success depends on how well it integrates its new tooling for novice developers. This paper will demonstrate and discuss where SwiftUI succeeds and fails at carving a new path for user interface development for new developers. This is done by comparisons against its existing imperative UI framework UIKit as well as elaborating on the background of SwiftUI and examples of how SwiftUI works to help developers. The paper will also discuss what exactly led to SwiftUI and how it is currently faring on Apple's latest operating systems. SwiftUI is a framework growing and evolving to serve the needs of 5 very different platforms with code that claims to be simpler to write and easier to deploy. The world of UI programming in iOS has been dominated by a Storyboard canvas for years, but SwiftUI claims to link this graphic-first development process with the code programmers are used to by keeping them side by side in constant sync. This bold move requires interactive programming capable of recompilation on the fly. As this paper will discuss, SwiftUI has garnered a community of developers giving it the main property it needs to succeed: a component library.

This project aims to incorporate the aspect of sentiment analysis into traditional stock analysis to enhance stock rating predictions by applying a reliance on the opinion of various stocks from the Internet. Headlines from eight major news publications and conversations from Yahoo! Finance’s “Conversations” feature were parsed through the Valence Aware Dictionary for Sentiment Reasoning (VADER) natural language processing package to determine numerical polarities which represented positivity or negativity for a given stock ticker. These generated polarities were paired with stock metrics typically observed by stock analysts as the feature set for a Logistic Regression machine learning model. The model was trained on roughly 1500 major stocks to determine a binary classification between a “Buy” or “Not Buy” rating for each stock, and the results of the model were inserted into the back-end of the Agora Web UI which emulates search engine behavior specifically for stocks found in NYSE and NASDAQ. The model reported an accuracy of 82.5% and for most major stocks, the model’s prediction correlated with stock analysts’ ratings. Given the volatility of the stock market and the propensity for hive-mind behavior in online forums, the performance of the Logistic Regression model would benefit from incorporating historical stock data and more sources of opinion to balance any subjectivity in the model.