Filtering by
- Creators: Computer Science and Engineering Program
- Creators: Nakamura, Mutsumi




Through my work with the Arizona State University Blockchain Research Lab (BRL) and JennyCo, one of the first Healthcare Information (HCI) HIPAA - compliant decentralized exchanges, I have had the opportunity to explore a unique cross-section of some of the most up and coming DLTs including both DAGs and blockchains. During this research, four major technologies (including JennyCo’s own systems) presented themselves as prime candidates for the comparative analysis of two models for implementing JennyCo’s system architecture for the monetization of healthcare information exchanges (HIEs). These four identified technologies and their underlying mechanisms will be explored thoroughly throughout the course of this paper and are listed with brief definitions as follows: Polygon - “Polygon is a “layer two” or “sidechain” scaling solution that runs alongside the Ethereum blockchain. MATIC is the network’s native cryptocurrency, which is used for fees, staking, and more” [8]. Polygon is the scalable layer involved in the L2SP architecture. Ethereum - “Ethereum is a decentralized blockchain platform that establishes a peer-to-peer network that securely executes and verifies application code, called smart contracts.” [9] This foundational Layer-1 runs thousands of nodes and creates a unique decentralized ecosystem governed by turing complete automated programs. Ethereum is the foundational Layer involved in the L2SP. Constellation - A novel Layer-0 data-centric peer-to-peer network that utilizes the “Hypergraph Transfer Protocol or HGTP, a DLT known as a [DAG] protocol with a novel reputation-based consensus model called Proof of Reputable Observation (PRO). Hypergraph is a feeless decentralized network that supports the transfer of $DAG cryptocurrency.” [10] JennyCo Protocol - Acts as a HIPAA compliant decentralized HIE by allowing consumers, big businesses, and brands to access and exchange user health data on a secure, interoperable, and accessible platform via DLT. The JennyCo Protocol implements utility tokens to reward buyers and sellers for exchanging data. Its protocol nature comes from its DLT implementation which governs the functioning of on-chain actions (e.g. smart contracts). In this case, these actions consist of secure and transparent health data exchange and monetization to reconstitute data ownership to those who generate that data [11]. With the direct experience of working closely with multiple companies behind the technologies listed, I have been exposed to the benefits and deficits of each of these technologies and their corresponding approaches. In this paper, I will use my experience with these technologies and their frameworks to explore two distributed ledger architecture protocols in order to determine the more effective model for implementing JennycCo’s health data exchange. I will begin this paper with an exploration of blockchain and directed acyclic graph (DAG) technologies to better understand their innate architectures and features. I will then move to an in-depth look at layered protocols, and healthcare data in the form of EHRs. Additionally, I will address the main challenges EHRs and HIEs face to present a deeper understanding of the challenges JennyCo is attempting to address. Finally, I will demonstrate my hypothesis: the Hypergraph Transfer Protocol (HGTP) model by Constellation presents significant advantages in scalability, interoperability, and external data security over the Layer-2 Scalability Protocol (L2SP) used by Polygon and Ethereum in implementing the JennyCo protocol. This will be done through a thorough breakdown of each protocol along with an analysis of relevant criteria including but not limited to: security, interoperability, and scalability. In doing so, I hope to determine the best framework for running JennyCo’s HIE Protocol.
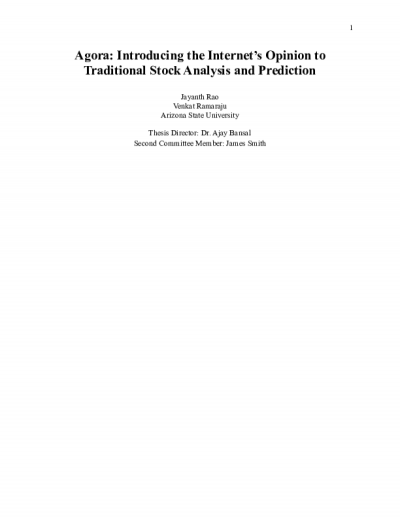
This project aims to incorporate the aspect of sentiment analysis into traditional stock analysis to enhance stock rating predictions by applying a reliance on the opinion of various stocks from the Internet. Headlines from eight major news publications and conversations from Yahoo! Finance’s “Conversations” feature were parsed through the Valence Aware Dictionary for Sentiment Reasoning (VADER) natural language processing package to determine numerical polarities which represented positivity or negativity for a given stock ticker. These generated polarities were paired with stock metrics typically observed by stock analysts as the feature set for a Logistic Regression machine learning model. The model was trained on roughly 1500 major stocks to determine a binary classification between a “Buy” or “Not Buy” rating for each stock, and the results of the model were inserted into the back-end of the Agora Web UI which emulates search engine behavior specifically for stocks found in NYSE and NASDAQ. The model reported an accuracy of 82.5% and for most major stocks, the model’s prediction correlated with stock analysts’ ratings. Given the volatility of the stock market and the propensity for hive-mind behavior in online forums, the performance of the Logistic Regression model would benefit from incorporating historical stock data and more sources of opinion to balance any subjectivity in the model.

This project aims to incorporate the aspect of sentiment analysis into traditional stock analysis to enhance stock rating predictions by applying a reliance on the opinion of various stocks from the Internet. Headlines from eight major news publications and conversations from Yahoo! Finance’s “Conversations” feature were parsed through the Valence Aware Dictionary for Sentiment Reasoning (VADER) natural language processing package to determine numerical polarities which represented positivity or negativity for a given stock ticker. These generated polarities were paired with stock metrics typically observed by stock analysts as the feature set for a Logistic Regression machine learning model. The model was trained on roughly 1500 major stocks to determine a binary classification between a “Buy” or “Not Buy” rating for each stock, and the results of the model were inserted into the back-end of the Agora Web UI which emulates search engine behavior specifically for stocks found in NYSE and NASDAQ. The model reported an accuracy of 82.5% and for most major stocks, the model’s prediction correlated with stock analysts’ ratings. Given the volatility of the stock market and the propensity for hive-mind behavior in online forums, the performance of the Logistic Regression model would benefit from incorporating historical stock data and more sources of opinion to balance any subjectivity in the model.

Since it doesn’t hurt to attempt to utilize feature extracted values to improve a model (if things don’t work out, one can always use their original features), the question may arise: how could the results of feature extraction on values such as sentiment affect a model’s ability to predict the movement of the stock market? This paper attempts to shine some light on to what the answer could be by deriving TextBlob sentiment values from Twitter data, and using Granger Causality Tests and logistic and linear regression to test if there exist a correlation or causation between the stock market and features extracted from public sentiment.

The e-commerce market utilizes information to target customers and drive business. More and more online services have become available, allowing consumers to make purchases and interact with an online system. For example, Amazon is one of the largest Internet-based retail companies. As people shop through this website, Amazon gathers huge amounts of data on its customers from personal information to shopping history to viewing history. After purchasing a product, the customer may leave reviews and give a rating based on their experience. Performing analytics on all of this data can provide insights into making more informed business and marketing decisions that can lead to business growth and also improve the customer experience.
For this thesis, I have trained binary classification models on a publicly available product review dataset from Amazon to predict whether a review has a positive or negative sentiment. The sentiment analysis process includes analyzing and encoding the human language, then extracting the sentiment from the resulting values. In the business world, sentiment analysis provides value by revealing insights into customer opinions and their behaviors. In this thesis, I will explain how to perform a sentiment analysis and analyze several different machine learning models. The algorithms for which I compared the results are KNN, Logistic Regression, Decision Trees, Random Forest, Naïve Bayes, Linear Support Vector Machines, and Support Vector Machines with an RBF kernel.