Filtering by
- Creators: Arizona State University. School of Sustainable Engineering and the Built Environment
- Creators: Cheng, Kewei

received increasing attention in recent years. The availability of sheer amounts of
user-generated data presents data scientists both opportunities and challenges. Opportunities are presented with additional data sources. The abundant link information
in social networks could provide another rich source in deriving implicit information
for social data mining. However, the vast majority of existing studies overwhelmingly
focus on positive links between users while negative links are also prevailing in real-
world social networks such as distrust relations in Epinions and foe links in Slashdot.
Though recent studies show that negative links have some added value over positive
links, it is dicult to directly employ them because of its distinct characteristics from
positive interactions. Another challenge is that label information is rather limited
in social media as the labeling process requires human attention and may be very
expensive. Hence, alternative criteria are needed to guide the learning process for
many tasks such as feature selection and sentiment analysis.
To address above-mentioned issues, I study two novel problems for signed social
networks mining, (1) unsupervised feature selection in signed social networks; and
(2) unsupervised sentiment analysis with signed social networks. To tackle the first problem, I propose a novel unsupervised feature selection framework SignedFS. In
particular, I model positive and negative links simultaneously for user preference
learning, and then embed the user preference learning into feature selection. To study the second problem, I incorporate explicit sentiment signals in textual terms and
implicit sentiment signals from signed social networks into a coherent model Signed-
Senti. Empirical experiments on real-world datasets corroborate the effectiveness of
these two frameworks on the tasks of feature selection and sentiment analysis.
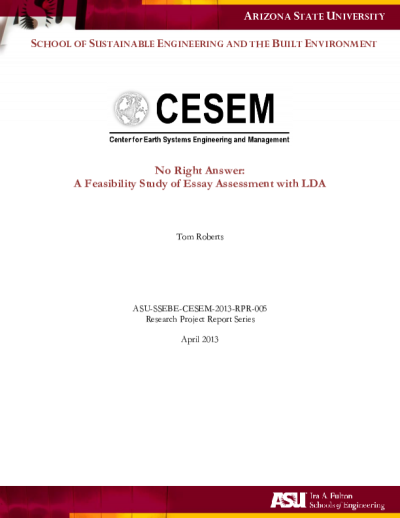
Essay scoring is a difficult and contentious business. The problem is exacerbated when there are no “right” answers for the essay prompts. This research developed a simple toolset for essay analysis by integrating a freely available Latent Dirichlet Allocation (LDA) implementation into a homegrown assessment assistant. The complexity of the essay assessment problem is demonstrated and illustrated with a representative collection of open-ended essays. This research also explores the use of “expert vectors” or “keyword essays” for maximizing the utility of LDA with small corpora. While, by itself, LDA appears insufficient for adequately scoring essays, it is quite capable of classifying responses to open-ended essay prompts and providing insight into the responses. This research also reports some trends that might be useful in scoring essays once more data is available. Some observations are made about these insights and a discussion of the use of LDA in qualitative assessment results in proposals that may assist other researchers in developing more complete essay assessment software.