Filtering by
- All Subjects: Declarative programming
- All Subjects: language modeling
- All Subjects: Web Scraping
- Creators: Bansal, Ajay
- Creators: Ramaraju, Venkat
- Creators: Henderson, Christopher

To facilitate rapid, correct, efficient, and intuitive development of graph based solutions we propose a new programming language construct - the search statement. Given a supra-root node, a procedure which determines the children of a given parent node, and optional definitions of the fail-fast acceptance or rejection of a solution, the search statement can conduct a search over any graph or network. Structurally, this statement is modelled after the common switch statement and is put into a largely imperative/procedural context to allow for immediate and intuitive development by most programmers. The Go programming language has been used as a foundation and proof-of-concept of the search statement. A Go compiler is provided which implements this construct.
The aim of this project is to understand the basic algorithmic components of the transformer deep learning architecture. At a high level, a transformer is a machine learning model based off of a recurrent neural network that adopts a self-attention mechanism, which can weigh significant parts of sequential input data which is very useful for solving problems in natural language processing and computer vision. There are other approaches to solving these problems which have been implemented in the past (i.e., convolutional neural networks and recurrent neural networks), but these architectures introduce the issue of the vanishing gradient problem when an input becomes too long (which essentially means the network loses its memory and halts learning) and have a slow training time in general. The transformer architecture’s features enable a much better “memory” and a faster training time, which makes it a more optimal architecture in solving problems. Most of this project will be spent producing a survey that captures the current state of research on the transformer, and any background material to understand it. First, I will do a keyword search of the most well cited and up-to-date peer reviewed publications on transformers to understand them conceptually. Next, I will investigate any necessary programming frameworks that will be required to implement the architecture. I will use this to implement a simplified version of the architecture or follow an easy to use guide or tutorial in implementing the architecture. Once the programming aspect of the architecture is understood, I will then Implement a transformer based on the academic paper “Attention is All You Need”. I will then slightly tweak this model using my understanding of the architecture to improve performance. Once finished, the details (i.e., successes, failures, process and inner workings) of the implementation will be evaluated and reported, as well as the fundamental concepts surveyed. The motivation behind this project is to explore the rapidly growing area of AI algorithms, and the transformer algorithm in particular was chosen because it is a major milestone for engineering with AI and software. Since their introduction, transformers have provided a very effective way of solving natural language processing, which has allowed any related applications to succeed with high speed while maintaining accuracy. Since then, this type of model can be applied to more cutting edge natural language processing applications, such as extracting semantic information from a text description and generating an image to satisfy it.

This thesis proposes a novel method of student modeling which uses pre-trained masked language models to model a student's reading comprehension abilities and detect words which are required for comprehension of a text. It explores the efficacy of using pre-trained masked language models to model human reading comprehension and presents a vocabulary study set generation pipeline using this method. This pipeline creates vocabulary study sets for explicit language learning that enable comprehension while still leaving some words to be acquired implicitly. Promising results show that masked language modeling can be used to model human comprehension and that the pipeline produces reasonably sized vocabulary study sets.
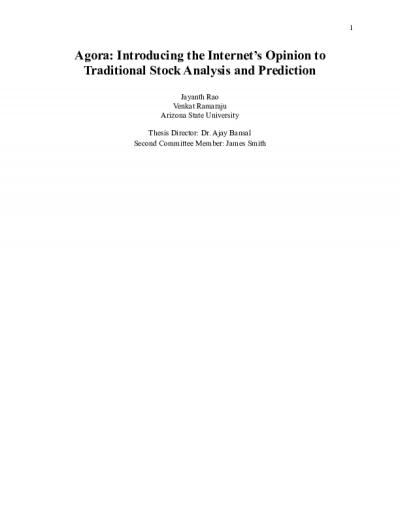
This project aims to incorporate the aspect of sentiment analysis into traditional stock analysis to enhance stock rating predictions by applying a reliance on the opinion of various stocks from the Internet. Headlines from eight major news publications and conversations from Yahoo! Finance’s “Conversations” feature were parsed through the Valence Aware Dictionary for Sentiment Reasoning (VADER) natural language processing package to determine numerical polarities which represented positivity or negativity for a given stock ticker. These generated polarities were paired with stock metrics typically observed by stock analysts as the feature set for a Logistic Regression machine learning model. The model was trained on roughly 1500 major stocks to determine a binary classification between a “Buy” or “Not Buy” rating for each stock, and the results of the model were inserted into the back-end of the Agora Web UI which emulates search engine behavior specifically for stocks found in NYSE and NASDAQ. The model reported an accuracy of 82.5% and for most major stocks, the model’s prediction correlated with stock analysts’ ratings. Given the volatility of the stock market and the propensity for hive-mind behavior in online forums, the performance of the Logistic Regression model would benefit from incorporating historical stock data and more sources of opinion to balance any subjectivity in the model.

User interface development on iOS is in a major transitionary state as Apple introduces a declarative and interactive framework called SwiftUI. SwiftUI’s success depends on how well it integrates its new tooling for novice developers. This paper will demonstrate and discuss where SwiftUI succeeds and fails at carving a new path for user interface development for new developers. This is done by comparisons against its existing imperative UI framework UIKit as well as elaborating on the background of SwiftUI and examples of how SwiftUI works to help developers. The paper will also discuss what exactly led to SwiftUI and how it is currently faring on Apple's latest operating systems. SwiftUI is a framework growing and evolving to serve the needs of 5 very different platforms with code that claims to be simpler to write and easier to deploy. The world of UI programming in iOS has been dominated by a Storyboard canvas for years, but SwiftUI claims to link this graphic-first development process with the code programmers are used to by keeping them side by side in constant sync. This bold move requires interactive programming capable of recompilation on the fly. As this paper will discuss, SwiftUI has garnered a community of developers giving it the main property it needs to succeed: a component library.

This project aims to incorporate the aspect of sentiment analysis into traditional stock analysis to enhance stock rating predictions by applying a reliance on the opinion of various stocks from the Internet. Headlines from eight major news publications and conversations from Yahoo! Finance’s “Conversations” feature were parsed through the Valence Aware Dictionary for Sentiment Reasoning (VADER) natural language processing package to determine numerical polarities which represented positivity or negativity for a given stock ticker. These generated polarities were paired with stock metrics typically observed by stock analysts as the feature set for a Logistic Regression machine learning model. The model was trained on roughly 1500 major stocks to determine a binary classification between a “Buy” or “Not Buy” rating for each stock, and the results of the model were inserted into the back-end of the Agora Web UI which emulates search engine behavior specifically for stocks found in NYSE and NASDAQ. The model reported an accuracy of 82.5% and for most major stocks, the model’s prediction correlated with stock analysts’ ratings. Given the volatility of the stock market and the propensity for hive-mind behavior in online forums, the performance of the Logistic Regression model would benefit from incorporating historical stock data and more sources of opinion to balance any subjectivity in the model.