Matching Items (47)

Description
Diseases have been part of human life for generations and evolve within the population, sometimes dying out while other times becoming endemic or the cause of recurrent outbreaks. The long term influence of a disease stems from different dynamics within or between pathogen-host, that have been analyzed and studied by many researchers using mathematical models. Co-infection with different pathogens is common, yet little is known about how infection with one pathogen affects the host's immunological response to another. Moreover, no work has been found in the literature that considers the variability of the host immune health or that examines a disease at the population level and its corresponding interconnectedness with the host immune system. Knowing that the spread of the disease in the population starts at the individual level, this thesis explores how variability in immune system response within an endemic environment affects an individual's vulnerability, and how prone it is to co-infections. Immunology-based models of Malaria and Tuberculosis (TB) are constructed by extending and modifying existing mathematical models in the literature. The two are then combined to give a single nine-variable model of co-infection with Malaria and TB. Because these models are difficult to gain any insight analytically due to the large number of parameters, a phenomenological model of co-infection is proposed with subsystems corresponding to the individual immunology-based model of a single infection. Within this phenomenological model, the variability of the host immune health is also incorporated through three different pathogen response curves using nonlinear bounded Michaelis-Menten functions that describe the level or state of immune system (healthy, moderate and severely compromised). The immunology-based models of Malaria and TB give numerical results that agree with the biological observations. The Malaria--TB co-infection model gives reasonable results and these suggest that the order in which the two diseases are introduced have an impact on the behavior of both. The subsystems of the phenomenological models that correspond to a single infection (either of Malaria or TB) mimic much of the observed behavior of the immunology-based counterpart and can demonstrate different behavior depending on the chosen pathogen response curve. In addition, varying some of the parameters and initial conditions in the phenomenological model yields a range of topologically different mathematical behaviors, which suggests that this behavior may be able to be observed in the immunology-based models as well. The phenomenological models clearly replicate the qualitative behavior of primary and secondary infection as well as co-infection. The mathematical solutions of the models correspond to the fundamental states described by immunologists: virgin state, immune state and tolerance state. The phenomenological model of co-infection also demonstrates a range of parameter values and initial conditions in which the introduction of a second disease causes both diseases to grow without bound even though those same parameters and initial conditions did not yield unbounded growth in the corresponding subsystems. This results applies to all three states of the host immune system. In terms of the immunology-based system, this would suggest the following: there may be parameter values and initial conditions in which a person can clear Malaria or TB (separately) from their system but in which the presence of both can result in the person dying of one of the diseases. Finally, this thesis studies links between epidemiology (population level) and immunology in an effort to assess the impact of pathogen's spread within the population on the immune response of individuals. Models of Malaria and TB are proposed that incorporate the immune system of the host into a mathematical model of an epidemic at the population level.
ContributorsSoho, Edmé L (Author) / Wirkus, Stephen (Thesis advisor) / Castillo-Chavez, Carlos (Thesis advisor) / Chowell-Puente, Gerardo (Committee member) / Arizona State University (Publisher)
Created2011

Description
In an effort to begin validating the large number of discovered candidate biomarkers, proteomics is beginning to shift from shotgun proteomic experiments towards targeted proteomic approaches that provide solutions to automation and economic concerns. Such approaches to validate biomarkers necessitate the mass spectrometric analysis of hundreds to thousands of human samples. As this takes place, a serendipitous opportunity has become evident. By the virtue that as one narrows the focus towards "single" protein targets (instead of entire proteomes) using pan-antibody-based enrichment techniques, a discovery science has emerged, so to speak. This is due to the largely unknown context in which "single" proteins exist in blood (i.e. polymorphisms, transcript variants, and posttranslational modifications) and hence, targeted proteomics has applications for established biomarkers. Furthermore, besides protein heterogeneity accounting for interferences with conventional immunometric platforms, it is becoming evident that this formerly hidden dimension of structural information also contains rich-pathobiological information. Consequently, targeted proteomics studies that aim to ascertain a protein's genuine presentation within disease- stratified populations and serve as a stepping-stone within a biomarker translational pipeline are of clinical interest. Roughly 128 million Americans are pre-diabetic, diabetic, and/or have kidney disease and public and private spending for treating these diseases is in the hundreds of billions of dollars. In an effort to create new solutions for the early detection and management of these conditions, described herein is the design, development, and translation of mass spectrometric immunoassays targeted towards diabetes and kidney disease. Population proteomics experiments were performed for the following clinically relevant proteins: insulin, C-peptide, RANTES, and parathyroid hormone. At least thirty-eight protein isoforms were detected. Besides the numerous disease correlations confronted within the disease-stratified cohorts, certain isoforms also appeared to be causally related to the underlying pathophysiology and/or have therapeutic implications. Technical advancements include multiplexed isoform quantification as well a "dual- extraction" methodology for eliminating non-specific proteins while simultaneously validating isoforms. Industrial efforts towards widespread clinical adoption are also described. Consequently, this work lays a foundation for the translation of mass spectrometric immunoassays into the clinical arena and simultaneously presents the most recent advancements concerning the mass spectrometric immunoassay approach.
ContributorsOran, Paul (Author) / Nelson, Randall (Thesis advisor) / Hayes, Mark (Thesis advisor) / Ros, Alexandra (Committee member) / Williams, Peter (Committee member) / Arizona State University (Publisher)
Created2011

Description
Solution methods for certain linear and nonlinear evolution equations are presented in this dissertation. Emphasis is placed mainly on the analytical treatment of nonautonomous differential equations, which are challenging to solve despite the existent numerical and symbolic computational software programs available. Ideas from the transformation theory are adopted allowing one to solve the problems under consideration from a non-traditional perspective. First, the Cauchy initial value problem is considered for a class of nonautonomous and inhomogeneous linear diffusion-type equation on the entire real line. Explicit transformations are used to reduce the equations under study to their corresponding standard forms emphasizing on natural relations with certain Riccati(and/or Ermakov)-type systems. These relations give solvability results for the Cauchy problem of the parabolic equation considered. The superposition principle allows to solve formally this problem from an unconventional point of view. An eigenfunction expansion approach is also considered for this general evolution equation. Examples considered to corroborate the efficacy of the proposed solution methods include the Fokker-Planck equation, the Black-Scholes model and the one-factor Gaussian Hull-White model. The results obtained in the first part are used to solve the Cauchy initial value problem for certain inhomogeneous Burgers-type equation. The connection between linear (the Diffusion-type) and nonlinear (Burgers-type) parabolic equations is stress in order to establish a strong commutative relation. Traveling wave solutions of a nonautonomous Burgers equation are also investigated. Finally, it is constructed explicitly the minimum-uncertainty squeezed states for quantum harmonic oscillators. They are derived by the action of corresponding maximal kinematical invariance group on the standard ground state solution. It is shown that the product of the variances attains the required minimum value only at the instances that one variance is a minimum and the other is a maximum, when the squeezing of one of the variances occurs. Such explicit construction is possible due to the relation between the diffusion-type equation studied in the first part and the time-dependent Schrodinger equation. A modication of the radiation field operators for squeezed photons in a perfect cavity is also suggested with the help of a nonstandard solution of Heisenberg's equation of motion.
ContributorsVega-Guzmán, José Manuel, 1982- (Author) / Sulov, Sergei K (Thesis advisor) / Castillo-Chavez, Carlos (Thesis advisor) / Platte, Rodrigo (Committee member) / Chowell-Puente, Gerardo (Committee member) / Arizona State University (Publisher)
Created2013

Description
Signal processing techniques have been used extensively in many engineering problems and in recent years its application has extended to non-traditional research fields such as biological systems. Many of these applications require extraction of a signal or parameter of interest from degraded measurements. One such application is mass spectrometry immunoassay (MSIA) which has been one of the primary methods of biomarker discovery techniques. MSIA analyzes protein molecules as potential biomarkers using time of flight mass spectrometry (TOF-MS). Peak detection in TOF-MS is important for biomarker analysis and many other MS related application. Though many peak detection algorithms exist, most of them are based on heuristics models. One of the ways of detecting signal peaks is by deploying stochastic models of the signal and noise observations. Likelihood ratio test (LRT) detector, based on the Neyman-Pearson (NP) lemma, is an uniformly most powerful test to decision making in the form of a hypothesis test. The primary goal of this dissertation is to develop signal and noise models for the electrospray ionization (ESI) TOF-MS data. A new method is proposed for developing the signal model by employing first principles calculations based on device physics and molecular properties. The noise model is developed by analyzing MS data from careful experiments in the ESI mass spectrometer. A non-flat baseline in MS data is common. The reasons behind the formation of this baseline has not been fully comprehended. A new signal model explaining the presence of baseline is proposed, though detailed experiments are needed to further substantiate the model assumptions. Signal detection schemes based on these signal and noise models are proposed. A maximum likelihood (ML) method is introduced for estimating the signal peak amplitudes. The performance of the detection methods and ML estimation are evaluated with Monte Carlo simulation which shows promising results. An application of these methods is proposed for fractional abundance calculation for biomarker analysis, which is mathematically robust and fundamentally different than the current algorithms. Biomarker panels for type 2 diabetes and cardiovascular disease are analyzed using existing MS analysis algorithms. Finally, a support vector machine based multi-classification algorithm is developed for evaluating the biomarkers' effectiveness in discriminating type 2 diabetes and cardiovascular diseases and is shown to perform better than a linear discriminant analysis based classifier.
ContributorsBuddi, Sai (Author) / Taylor, Thomas (Thesis advisor) / Cochran, Douglas (Thesis advisor) / Nelson, Randall (Committee member) / Duman, Tolga (Committee member) / Arizona State University (Publisher)
Created2012

Description
Cancer claims hundreds of thousands of lives every year in US alone. Finding ways for early detection of cancer onset is crucial for better management and treatment of cancer. Thus, biomarkers especially protein biomarkers, being the functional units which reflect dynamic physiological changes, need to be discovered. Though important, there are only a few approved protein cancer biomarkers till date. To accelerate this process, fast, comprehensive and affordable assays are required which can be applied to large population studies. For this, these assays should be able to comprehensively characterize and explore the molecular diversity of nominally "single" proteins across populations. This information is usually unavailable with commonly used immunoassays such as ELISA (enzyme linked immunosorbent assay) which either ignore protein microheterogeneity, or are confounded by it. To this end, mass spectrometric immuno assays (MSIA) for three different human plasma proteins have been developed. These proteins viz. IGF-1, hemopexin and tetranectin have been found in reported literature to show correlations with many diseases along with several carcinomas. Developed assays were used to extract entire proteins from plasma samples and subsequently analyzed on mass spectrometric platforms. Matrix assisted laser desorption ionization (MALDI) and electrospray ionization (ESI) mass spectrometric techniques where used due to their availability and suitability for the analysis. This resulted in visibility of different structural forms of these proteins showing their structural micro-heterogeneity which is invisible to commonly used immunoassays. These assays are fast, comprehensive and can be applied in large sample studies to analyze proteins for biomarker discovery.
ContributorsRai, Samita (Author) / Nelson, Randall (Thesis advisor) / Hayes, Mark (Thesis advisor) / Borges, Chad (Committee member) / Ros, Alexandra (Committee member) / Arizona State University (Publisher)
Created2012

Description
Mortality of 1918 influenza virus was high, partly due to bacteria coinfections. We characterize pandemic mortality in Arizona, which had high prevalence of tuberculosis. We applied regressions to over 35,000 data points to estimate the basic reproduction number and excess mortality. Age-specific mortality curves show elevated mortality for all age groups, especially the young, and senior sparing effects. The low value for reproduction number indicates that transmissibility was moderately low.
ContributorsJenner, Melinda Eva (Author) / Chowell-Puente, Gerardo (Thesis director) / Kostelich, Eric (Committee member) / Barrett, The Honors College (Contributor) / School of Mathematical and Statistical Sciences (Contributor) / School of Life Sciences (Contributor)
Created2015-05

Description
Background: While research has quantified the mortality burden of the 1957 H2N2 influenza pandemic in the United States, little is known about how the virus spread locally in Arizona, an area where the dry climate was promoted as reducing respiratory illness transmission yet tuberculosis prevalence was high.
Methods: Using archival death certificates from 1954 to 1961, this study quantified the age-specific seasonal patterns, excess-mortality rates, and transmissibility patterns of the 1957 pandemic in Maricopa County, Arizona. By applying cyclical Serfling linear regression models to weekly mortality rates, the excess-mortality rates due to respiratory and all-causes were estimated for each age group during the pandemic period. The reproduction number was quantified from weekly data using a simple growth rate method and generation intervals of 3 and 4 days. Local newspaper articles from The Arizona Republic were analyzed from 1957-1958.
Results: Excess-mortality rates varied between waves, age groups, and causes of death, but overall remained low. From October 1959-June 1960, the most severe wave of the pandemic, the absolute excess-mortality rate based on respiratory deaths per 10,000 population was 17.85 in the elderly (≥65 years). All other age groups had extremely low excess-mortality and the typical U-shaped age-pattern was absent. However, relative risk was greatest (3.61) among children and young adolescents (5-14 years) from October 1957-March 1958, based on incidence rates of respiratory deaths. Transmissibility was greatest during the same 1957-1958 period, when the mean reproduction number was 1.08-1.11, assuming 3 or 4 day generation intervals and exponential or fixed distributions.
Conclusions: Maricopa County largely avoided pandemic influenza from 1957-1961. Understanding this historical pandemic and the absence of high excess-mortality rates and transmissibility in Maricopa County may help public health officials prepare for and mitigate future outbreaks of influenza.
Methods: Using archival death certificates from 1954 to 1961, this study quantified the age-specific seasonal patterns, excess-mortality rates, and transmissibility patterns of the 1957 pandemic in Maricopa County, Arizona. By applying cyclical Serfling linear regression models to weekly mortality rates, the excess-mortality rates due to respiratory and all-causes were estimated for each age group during the pandemic period. The reproduction number was quantified from weekly data using a simple growth rate method and generation intervals of 3 and 4 days. Local newspaper articles from The Arizona Republic were analyzed from 1957-1958.
Results: Excess-mortality rates varied between waves, age groups, and causes of death, but overall remained low. From October 1959-June 1960, the most severe wave of the pandemic, the absolute excess-mortality rate based on respiratory deaths per 10,000 population was 17.85 in the elderly (≥65 years). All other age groups had extremely low excess-mortality and the typical U-shaped age-pattern was absent. However, relative risk was greatest (3.61) among children and young adolescents (5-14 years) from October 1957-March 1958, based on incidence rates of respiratory deaths. Transmissibility was greatest during the same 1957-1958 period, when the mean reproduction number was 1.08-1.11, assuming 3 or 4 day generation intervals and exponential or fixed distributions.
Conclusions: Maricopa County largely avoided pandemic influenza from 1957-1961. Understanding this historical pandemic and the absence of high excess-mortality rates and transmissibility in Maricopa County may help public health officials prepare for and mitigate future outbreaks of influenza.
ContributorsCobos, April J (Author) / Jehn, Megan (Thesis director) / Chowell-Puente, Gerardo (Committee member) / Barrett, The Honors College (Contributor) / School of Human Evolution and Social Change (Contributor) / School of Life Sciences (Contributor)
Created2015-05
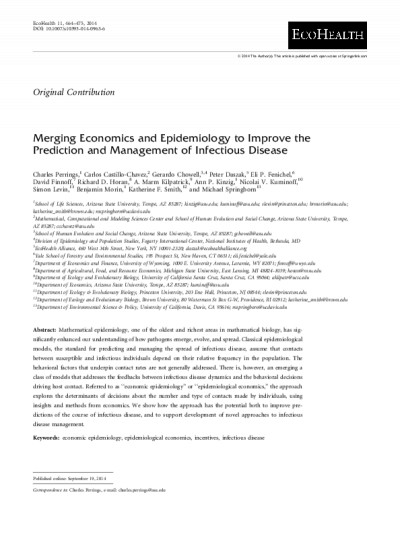
Description
Mathematical epidemiology, one of the oldest and richest areas in mathematical biology, has significantly enhanced our understanding of how pathogens emerge, evolve, and spread. Classical epidemiological models, the standard for predicting and managing the spread of infectious disease, assume that contacts between susceptible and infectious individuals depend on their relative frequency in the population. The behavioral factors that underpin contact rates are not generally addressed. There is, however, an emerging a class of models that addresses the feedbacks between infectious disease dynamics and the behavioral decisions driving host contact. Referred to as “economic epidemiology” or “epidemiological economics,” the approach explores the determinants of decisions about the number and type of contacts made by individuals, using insights and methods from economics. We show how the approach has the potential both to improve predictions of the course of infectious disease, and to support development of novel approaches to infectious disease management.
ContributorsPerrings, Charles (Author) / Castillo-Chavez, Carlos (Author) / Chowell-Puente, Gerardo (Author) / Daszak, Peter (Author) / Fenichel, Eli P. (Author) / Finnoff, David (Author) / Horan, Richard D. (Author) / Kilpatrick, A. Marm (Author) / Kinzig, Ann (Author) / Kuminoff, Nicolai (Author) / Levin, Simon (Author) / Morin, Benjamin (Author) / Smith, Katherine F. (Author) / Springborn, Michael (Author) / Simon M. Levin Mathematical, Computational and Modeling Sciences Center (Contributor) / College of Liberal Arts and Sciences (Contributor) / School of Life Sciences (Contributor) / School of Human Evolution and Social Change (Contributor) / W.P. Carey School of Business (Contributor) / Economics (Contributor) / Julie Ann Wrigley Global Institute of Sustainability (Contributor)
Created2015-12-01
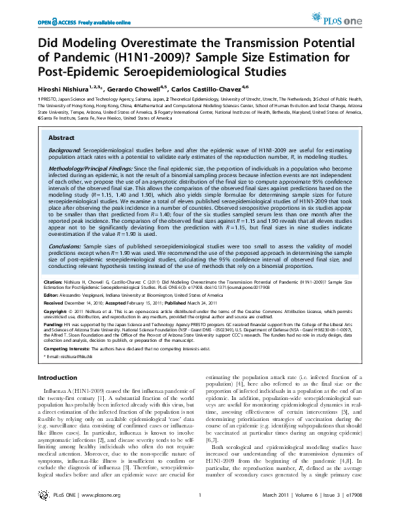
Description
Background
Seroepidemiological studies before and after the epidemic wave of H1N1-2009 are useful for estimating population attack rates with a potential to validate early estimates of the reproduction number, R, in modeling studies.
Methodology/Principal Findings
Since the final epidemic size, the proportion of individuals in a population who become infected during an epidemic, is not the result of a binomial sampling process because infection events are not independent of each other, we propose the use of an asymptotic distribution of the final size to compute approximate 95% confidence intervals of the observed final size. This allows the comparison of the observed final sizes against predictions based on the modeling study (R = 1.15, 1.40 and 1.90), which also yields simple formulae for determining sample sizes for future seroepidemiological studies. We examine a total of eleven published seroepidemiological studies of H1N1-2009 that took place after observing the peak incidence in a number of countries. Observed seropositive proportions in six studies appear to be smaller than that predicted from R = 1.40; four of the six studies sampled serum less than one month after the reported peak incidence. The comparison of the observed final sizes against R = 1.15 and 1.90 reveals that all eleven studies appear not to be significantly deviating from the prediction with R = 1.15, but final sizes in nine studies indicate overestimation if the value R = 1.90 is used.
Conclusions
Sample sizes of published seroepidemiological studies were too small to assess the validity of model predictions except when R = 1.90 was used. We recommend the use of the proposed approach in determining the sample size of post-epidemic seroepidemiological studies, calculating the 95% confidence interval of observed final size, and conducting relevant hypothesis testing instead of the use of methods that rely on a binomial proportion.
Seroepidemiological studies before and after the epidemic wave of H1N1-2009 are useful for estimating population attack rates with a potential to validate early estimates of the reproduction number, R, in modeling studies.
Methodology/Principal Findings
Since the final epidemic size, the proportion of individuals in a population who become infected during an epidemic, is not the result of a binomial sampling process because infection events are not independent of each other, we propose the use of an asymptotic distribution of the final size to compute approximate 95% confidence intervals of the observed final size. This allows the comparison of the observed final sizes against predictions based on the modeling study (R = 1.15, 1.40 and 1.90), which also yields simple formulae for determining sample sizes for future seroepidemiological studies. We examine a total of eleven published seroepidemiological studies of H1N1-2009 that took place after observing the peak incidence in a number of countries. Observed seropositive proportions in six studies appear to be smaller than that predicted from R = 1.40; four of the six studies sampled serum less than one month after the reported peak incidence. The comparison of the observed final sizes against R = 1.15 and 1.90 reveals that all eleven studies appear not to be significantly deviating from the prediction with R = 1.15, but final sizes in nine studies indicate overestimation if the value R = 1.90 is used.
Conclusions
Sample sizes of published seroepidemiological studies were too small to assess the validity of model predictions except when R = 1.90 was used. We recommend the use of the proposed approach in determining the sample size of post-epidemic seroepidemiological studies, calculating the 95% confidence interval of observed final size, and conducting relevant hypothesis testing instead of the use of methods that rely on a binomial proportion.
ContributorsNishiura, Hiroshi (Author) / Chowell-Puente, Gerardo (Author) / Castillo-Chavez, Carlos (Author) / Simon M. Levin Mathematical, Computational and Modeling Sciences Center (Contributor) / College of Liberal Arts and Sciences (Contributor) / School of Human Evolution and Social Change (Contributor)
Created2011-03-24

Description
Massive glycerol cluster ions with many charges (~ 106 Da, ~ ±100 charges) have been generated by electrospray to bombard biomolecules and biological sample surfaces. The low impact energy per nucleon facilitates intact sputtering and ionization of biomolecules which can be subsequently imaged. Various lipids, peptides and proteins have been studied. The primary cluster ion source has been coupled with an ion-microscope imaging mass spectrometer (TRIFT-1, Physical Electronics). A lateral resolution of ~3µm has been demonstrated, which is acceptable for sub-cellular imaging of animal cells (e.g. single cancer cell imaging in early diagnosis). Since the available amount of target molecules per pixel is limited in biological samples, the measurement of useful ion yields (ratio of detected molecular ion counts to the sample molecules sputtered) is important to determine whether enough ion counts per pixel can be obtained. The useful ion yields of several lipids and peptides are in the 1-3×10-5 range. A 3×3 µm2lipid bilayer can produce ~260 counts/pixel for a meaningful 3×3 µm2 pixel ion image. This method can probably be used in cell imaging in the future, when there is a change in the lipid contents of the cell membrane (e.g. cancer cells vs. normal cells).
ContributorsZhang, Jitao (Author) / Williams, Peter (Thesis advisor) / Hayes, Mark (Committee member) / Nelson, Randall (Committee member) / Arizona State University (Publisher)
Created2015