ASU Scholarship Showcase
This growing collection consists of scholarly works authored by ASU-affiliated faculty, staff, and community members, and it contains many open access articles. ASU-affiliated authors are encouraged to Share Your Work in KEEP.
Filtering by
- All Subjects: regularization parameter estimation
- All Subjects: research computing and data
- Language: English
- Resource Type: Text
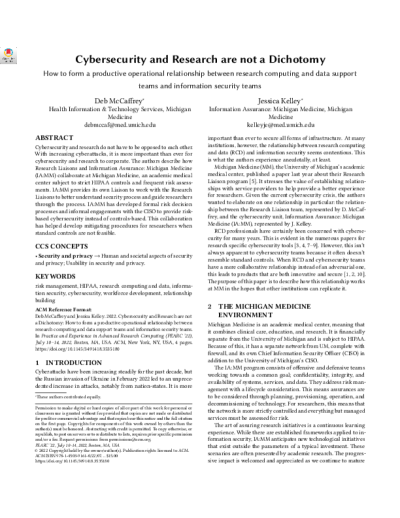

Tikhonov regularization for projected solutions of large-scale ill-posed problems is considered. The Golub{Kahan iterative bidiagonalization is used to project the problem onto a subspace and regularization then applied to nd a subspace approximation to the full problem. Determination of the regularization, parameter for the projected problem by unbiased predictive risk estimation, generalized cross validation, and discrepancy principle techniques is investigated. It is shown that the regularized parameter obtained by the unbiased predictive risk estimator can provide a good estimate which can be used for a full problem that is moderately to severely ill-posed. A similar analysis provides the weight parameter for the weighted generalized cross validation such that the approach is also useful in these cases, and also explains why the generalized cross validation without weighting is not always useful. All results are independent of whether systems are over- or underdetermined. Numerical simulations for standard one-dimensional test problems and two- dimensional data, for both image restoration and tomographic image reconstruction, support the analysis and validate the techniques. The size of the projected problem is found using an extension of a noise revealing function for the projected problem [I. Hn etynkov a, M. Ple singer, and Z. Strako s, BIT Numer. Math., 49 (2009), pp. 669{696]. Furthermore, an iteratively reweighted regularization approach for edge preserving regularization is extended for projected systems, providing stabilization of the solutions of the projected systems and reducing dependence on the determination of the size of the projected subspace.