Matching Items (77)
Description
Original exhibit panel text and an associated interview with ASU faculty Charles Backus and Harvey Bryan for the exhibit presented at the Luhrs Gallery, Hayden Library, Fall, 2013.
ContributorsSpindler, Rob (Curator) / Backus, Charles (Interviewee) / Bryan, Harvey (Interviewee)
Created2013-07-01
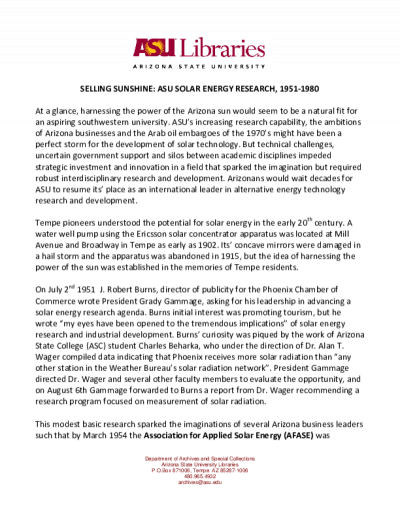
ContributorsSpindler, Rob (Curator) / Backus, Charles (Interviewee) / Bryan, Harvey (Interviewee)
Created2013-07-01
ContributorsSpindler, Rob (Curator) / Backus, Charles (Interviewee) / Bryan, Harvey (Interviewee)
Created2013-07-01

Description
Accounting for over a third of all emerging and re-emerging infections, viruses represent a major public health threat, which researchers and epidemiologists across the world have been attempting to contain for decades. Recently, genomics-based surveillance of viruses through methods such as virus phylogeography has grown into a popular tool for infectious disease monitoring. When conducting such surveillance studies, researchers need to manually retrieve geographic metadata denoting the location of infected host (LOIH) of viruses from public sequence databases such as GenBank and any publication related to their study. The large volume of semi-structured and unstructured information that must be reviewed for this task, along with the ambiguity of geographic locations, make it especially challenging. Prior work has demonstrated that the majority of GenBank records lack sufficient geographic granularity concerning the LOIH of viruses. As a result, reviewing full-text publications is often necessary for conducting in-depth analysis of virus migration, which can be a very time-consuming process. Moreover, integrating geographic metadata pertaining to the LOIH of viruses from different sources, including different fields in GenBank records as well as full-text publications, and normalizing the integrated metadata to unique identifiers for subsequent analysis, are also challenging tasks, often requiring expert domain knowledge. Therefore, automated information extraction (IE) methods could help significantly accelerate this process, positively impacting public health research. However, very few research studies have attempted the use of IE methods in this domain.
This work explores the use of novel knowledge-driven geographic IE heuristics for extracting, integrating, and normalizing the LOIH of viruses based on information available in GenBank and related publications; when evaluated on manually annotated test sets, the methods were found to have a high accuracy and shown to be adequate for addressing this challenging problem. It also presents GeoBoost, a pioneering software system for georeferencing GenBank records, as well as a large-scale database containing over two million virus GenBank records georeferenced using the algorithms introduced here. The methods, database and software developed here could help support diverse public health domains focusing on sequence-informed virus surveillance, thereby enhancing existing platforms for controlling and containing disease outbreaks.
This work explores the use of novel knowledge-driven geographic IE heuristics for extracting, integrating, and normalizing the LOIH of viruses based on information available in GenBank and related publications; when evaluated on manually annotated test sets, the methods were found to have a high accuracy and shown to be adequate for addressing this challenging problem. It also presents GeoBoost, a pioneering software system for georeferencing GenBank records, as well as a large-scale database containing over two million virus GenBank records georeferenced using the algorithms introduced here. The methods, database and software developed here could help support diverse public health domains focusing on sequence-informed virus surveillance, thereby enhancing existing platforms for controlling and containing disease outbreaks.
ContributorsTahsin, Tasnia (Author) / Gonzalez, Graciela (Thesis advisor) / Scotch, Matthew (Thesis advisor) / Runger, George C. (Committee member) / Arizona State University (Publisher)
Created2019

Description
The aim of this research study is to develop a passive architectural design morphology, tuned to the Sonoran Desert, which redefines Desert Modernism and integrates: (a) mitigation of heat transfer through the exterior envelope, and (b) use of daylight to inform appropriate architectural massing. The research investigation was delimited to mid-nineteenth century European modernist examples, and ends with mid-twentieth century modern architecture in the southwestern United States as viewed through the lens of environmental design. The specific focus was on Desert Modernism, a quasi-architectural movement, which purportedly had its beginnings in 1923 with the Coachella Valley, Popinoe Desert Cabin.
A mixed-method research strategy comprised of interpretive-historical research, virtual simulation/modeling analysis and logical argumentation is used. Succinct discussions on desert vernacular design, Modernism’s global propagation, and the International Style reinterpretations were illustrated to introduce the possibility of a relationship between Modernism and the vernacular. A directed examination of climatic responses included within examples of California Modernism, the Case Study Houses and Desert Modernism follows. Three case studies: a) the Frey House II, b) the Triad Apartments, and c) the Analemma House were assessed using virtual simulation and mathematical calculations, to provide conclusive results on the relevance of regionally tuned exterior envelope design and planning tactics for the Phoenix, Arizona area. Together, these findings suggest a correlation between environmental design principles, vernacular architecture, and Modernism.
A mixed-method research strategy comprised of interpretive-historical research, virtual simulation/modeling analysis and logical argumentation is used. Succinct discussions on desert vernacular design, Modernism’s global propagation, and the International Style reinterpretations were illustrated to introduce the possibility of a relationship between Modernism and the vernacular. A directed examination of climatic responses included within examples of California Modernism, the Case Study Houses and Desert Modernism follows. Three case studies: a) the Frey House II, b) the Triad Apartments, and c) the Analemma House were assessed using virtual simulation and mathematical calculations, to provide conclusive results on the relevance of regionally tuned exterior envelope design and planning tactics for the Phoenix, Arizona area. Together, these findings suggest a correlation between environmental design principles, vernacular architecture, and Modernism.
ContributorsSoltero, Ed (Author) / Zygas, Kestutis (Thesis advisor) / Bryan, Harvey (Thesis advisor) / Domin, Christopher (Committee member) / Arizona State University (Publisher)
Created2019

Description
In conventional supervised learning tasks, information retrieval from extensive collections of data happens automatically at low cost, whereas in many real-world problems obtaining labeled data can be hard, time-consuming, and expensive. Consider healthcare systems, for example, where unlabeled medical images are abundant while labeling requires a considerable amount of knowledge from experienced physicians. Active learning addresses this challenge with an iterative process to select instances from the unlabeled data to annotate and improve the supervised learner. At each step, the query of examples to be labeled can be considered as a dilemma between exploitation of the supervised learner's current knowledge and exploration of the unlabeled input features.
Motivated by the need for efficient active learning strategies, this dissertation proposes new algorithms for batch-mode, pool-based active learning. The research considers the following questions: how can unsupervised knowledge of the input features (exploration) improve learning when incorporated with supervised learning (exploitation)? How to characterize exploration in active learning when data is high-dimensional? Finally, how to adaptively make a balance between exploration and exploitation?
The first contribution proposes a new active learning algorithm, Cluster-based Stochastic Query-by-Forest (CSQBF), which provides a batch-mode strategy that accelerates learning with added value from exploration and improved exploitation scores. CSQBF balances exploration and exploitation using a probabilistic scoring criterion based on classification probabilities from a tree-based ensemble model within each data cluster.
The second contribution introduces two more query strategies, Double Margin Active Learning (DMAL) and Cluster Agnostic Active Learning (CAAL), that combine consistent exploration and exploitation modules into a coherent and unified measure for label query. Instead of assuming a fixed clustering structure, CAAL and DMAL adopt a soft-clustering strategy which provides a new approach to formalize exploration in active learning.
The third contribution addresses the challenge of dynamically making a balance between exploration and exploitation criteria throughout the active learning process. Two adaptive algorithms are proposed based on feedback-driven bandit optimization frameworks that elegantly handle this issue by learning the relationship between exploration-exploitation trade-off and an active learner's performance.
Motivated by the need for efficient active learning strategies, this dissertation proposes new algorithms for batch-mode, pool-based active learning. The research considers the following questions: how can unsupervised knowledge of the input features (exploration) improve learning when incorporated with supervised learning (exploitation)? How to characterize exploration in active learning when data is high-dimensional? Finally, how to adaptively make a balance between exploration and exploitation?
The first contribution proposes a new active learning algorithm, Cluster-based Stochastic Query-by-Forest (CSQBF), which provides a batch-mode strategy that accelerates learning with added value from exploration and improved exploitation scores. CSQBF balances exploration and exploitation using a probabilistic scoring criterion based on classification probabilities from a tree-based ensemble model within each data cluster.
The second contribution introduces two more query strategies, Double Margin Active Learning (DMAL) and Cluster Agnostic Active Learning (CAAL), that combine consistent exploration and exploitation modules into a coherent and unified measure for label query. Instead of assuming a fixed clustering structure, CAAL and DMAL adopt a soft-clustering strategy which provides a new approach to formalize exploration in active learning.
The third contribution addresses the challenge of dynamically making a balance between exploration and exploitation criteria throughout the active learning process. Two adaptive algorithms are proposed based on feedback-driven bandit optimization frameworks that elegantly handle this issue by learning the relationship between exploration-exploitation trade-off and an active learner's performance.
ContributorsShams, Ghazal (Author) / Runger, George C. (Thesis advisor) / Montgomery, Douglas C. (Committee member) / Escobedo, Adolfo (Committee member) / Pedrielli, Giulia (Committee member) / Arizona State University (Publisher)
Created2020

Description
The Cognitive Decision Support (CDS) model is proposed. The model is widely applicable and scales to realistic, complex decision problems based on adaptive learning. The utility of a decision is discussed and four types of decisions associated with CDS model are identified. The CDS model is designed to learn decision utilities. Data enrichment is introduced to promote the effectiveness of learning. Grouping is introduced for large-scale decision learning. Introspection and adjustment are presented for adaptive learning. Triage recommendation is incorporated to indicate the trustworthiness of suggested decisions.
The CDS model and methodologies are integrated into an architecture using concepts from cognitive computing. The proposed architecture is implemented with an example use case to inventory management.
Reinforcement learning (RL) is discussed as an alternative, generalized adaptive learning engine for the CDS system to handle the complexity of many problems with unknown environments. An adaptive state dimension with context that can increase with newly available information is discussed. Several enhanced components for RL which are critical for complex use cases are integrated. Deep Q networks are embedded with the adaptive learning methodologies and applied to an example supply chain management problem on capacity planning.
A new approach using Ito stochastic processes is proposed as a more generalized method to generate non-stationary demands in various patterns that can be used in decision problems. The proposed method generates demands with varying non-stationary patterns, including trend, cyclical, seasonal, and irregular patterns. Conventional approaches are identified as special cases of the proposed method. Demands are illustrated in realistic settings for various decision models. Various statistical criteria are applied to filter the generated demands. The method is applied to a real-world example.
The CDS model and methodologies are integrated into an architecture using concepts from cognitive computing. The proposed architecture is implemented with an example use case to inventory management.
Reinforcement learning (RL) is discussed as an alternative, generalized adaptive learning engine for the CDS system to handle the complexity of many problems with unknown environments. An adaptive state dimension with context that can increase with newly available information is discussed. Several enhanced components for RL which are critical for complex use cases are integrated. Deep Q networks are embedded with the adaptive learning methodologies and applied to an example supply chain management problem on capacity planning.
A new approach using Ito stochastic processes is proposed as a more generalized method to generate non-stationary demands in various patterns that can be used in decision problems. The proposed method generates demands with varying non-stationary patterns, including trend, cyclical, seasonal, and irregular patterns. Conventional approaches are identified as special cases of the proposed method. Demands are illustrated in realistic settings for various decision models. Various statistical criteria are applied to filter the generated demands. The method is applied to a real-world example.
ContributorsKee, Seho (Author) / Runger, George C. (Thesis advisor) / Escobedo, Adolfo (Committee member) / Gel, Esma (Committee member) / Janakiram, Mani (Committee member) / Rogers, Dale (Committee member) / Arizona State University (Publisher)
Created2020

Description
One of the main challenges in testing artificial intelligence (AI) enabled cyber physicalsystems (CPS) such as autonomous driving systems and internet-of-things (IoT) medical
devices is the presence of machine learning components, for which formal properties are
difficult to establish. In addition, operational components interaction circumstances, inclusion of human-in-the-loop, and environmental changes result in a myriad of safety concerns
all of which may not only be comprehensibly tested before deployment but also may not
even have been detected during design and testing phase. This dissertation identifies major challenges of safety verification of AI-enabled safety critical systems and addresses the
safety problem by proposing an operational safety verification technique which relies on
solving the following subproblems:
1. Given Input/Output operational traces collected from sensors/actuators, automatically
learn a hybrid automata (HA) representation of the AI-enabled CPS.
2. Given the learned HA, evaluate the operational safety of AI-enabled CPS in the field.
This dissertation presents novel approaches for learning hybrid automata model from time
series traces collected from the operation of the AI-enabled CPS in the real world for linear
and nonlinear CPS. The learned model allows operational safety to be stringently evaluated
by comparing the learned HA model against a reference specifications model of the system.
The proposed techniques are evaluated on the artificial pancreas control system
devices is the presence of machine learning components, for which formal properties are
difficult to establish. In addition, operational components interaction circumstances, inclusion of human-in-the-loop, and environmental changes result in a myriad of safety concerns
all of which may not only be comprehensibly tested before deployment but also may not
even have been detected during design and testing phase. This dissertation identifies major challenges of safety verification of AI-enabled safety critical systems and addresses the
safety problem by proposing an operational safety verification technique which relies on
solving the following subproblems:
1. Given Input/Output operational traces collected from sensors/actuators, automatically
learn a hybrid automata (HA) representation of the AI-enabled CPS.
2. Given the learned HA, evaluate the operational safety of AI-enabled CPS in the field.
This dissertation presents novel approaches for learning hybrid automata model from time
series traces collected from the operation of the AI-enabled CPS in the real world for linear
and nonlinear CPS. The learned model allows operational safety to be stringently evaluated
by comparing the learned HA model against a reference specifications model of the system.
The proposed techniques are evaluated on the artificial pancreas control system
ContributorsLamrani, Imane (Author) / Gupta, Sandeep Ks (Thesis advisor) / Banerjee, Ayan (Committee member) / Zhang, Yi (Committee member) / Runger, George C. (Committee member) / Rodriguez, Armando (Committee member) / Arizona State University (Publisher)
Created2020

Description
All biological processes like cell growth, cell differentiation, development, and aging requires a series of steps which are characterized by gene regulation. Studies have shown that gene regulation is the key to various traits and diseases. Various factors affect the gene regulation which includes genetic signals, epigenetic tracks, genetic variants, etc. Deciphering and cataloging these functional genetic elements in the non-coding regions of the genome is one of the biggest challenges in precision medicine and genetic research. This thesis presents two different approaches to identifying these elements: TreeMap and DeepCORE. The first approach involves identifying putative causal genetic variants in cis-eQTL accounting for multisite effects and genetic linkage at a locus. TreeMap performs an organized search for individual and multiple causal variants using a tree guided nested machine learning method. DeepCORE on the other hand explores novel deep learning techniques that models the relationship between genetic, epigenetic and transcriptional patterns across tissues and cell lines and identifies co-operative regulatory elements that affect gene regulation. These two methods are believed to be the link for genotype-phenotype association and a necessary step to explaining various complex diseases and missing heritability.
ContributorsChandrashekar, Pramod Bharadwaj (Author) / Liu, Li (Thesis advisor) / Runger, George C. (Committee member) / Dinu, Valentin (Committee member) / Arizona State University (Publisher)
Created2020

Description
In 2018, building energy use accounted for over 40% of total primary energy consumption in the United States; moreover, buildings account for ~40% of national CO2 emissions. One method for curbing energy use in buildings is to apply Demand Side Management (DSM) strategies, which focus on reducing the energy demand through various technological and operational approaches in different building sectors.
This PhD research examines the integration of DSM strategies in existing residential and commercial buildings in the Phoenix, Arizona metropolitan area, a hot-arid climate. The author proposes three different case studies to evaluate the effectiveness of one DSM strategy in buildings, namely the integration of Phase Change Materials (PCMs). PCMs store energy in the freezing process and use that stored energy in the melting process to reduce the energy demand. The goal of these case studies is to analyze the potential of each strategy to reduce peak load and overall energy consumption in existing buildings.
First, this dissertation discusses the efficacy of coupling PCMs with precooling strategies in residential buildings to reduce peak demand. The author took a case study approach and simulated two precooling strategies, with and without PCM integration, in two sample single-family homes to assess the impact of the DSM strategies (i.e., precooling and PCM integration) on load shifting and load shedding in each home.
Second, this research addresses the feasibility of using PCMs as sensible and latent heat storage in commercial buildings. The author documents the process of choosing buildings for PCM installation, as well as the selection of PCMs for retrofitting purposes. Commercial building case studies compare experimental and simulation results, focusing on the impact of the PCMs on reducing the total annual energy demand and energy cost.
Finally, this research proposes a novel process for selecting PCMs as energy efficiency measures for building retrofits. This process facilitates the selection of a building and PCM that are complementary. Implementation of this process has not yet been tested; however, the process was developed based on experimental and simulation results from prior studies, and it would alleviate many of the PCM performance issues documented in those studies.
This PhD research examines the integration of DSM strategies in existing residential and commercial buildings in the Phoenix, Arizona metropolitan area, a hot-arid climate. The author proposes three different case studies to evaluate the effectiveness of one DSM strategy in buildings, namely the integration of Phase Change Materials (PCMs). PCMs store energy in the freezing process and use that stored energy in the melting process to reduce the energy demand. The goal of these case studies is to analyze the potential of each strategy to reduce peak load and overall energy consumption in existing buildings.
First, this dissertation discusses the efficacy of coupling PCMs with precooling strategies in residential buildings to reduce peak demand. The author took a case study approach and simulated two precooling strategies, with and without PCM integration, in two sample single-family homes to assess the impact of the DSM strategies (i.e., precooling and PCM integration) on load shifting and load shedding in each home.
Second, this research addresses the feasibility of using PCMs as sensible and latent heat storage in commercial buildings. The author documents the process of choosing buildings for PCM installation, as well as the selection of PCMs for retrofitting purposes. Commercial building case studies compare experimental and simulation results, focusing on the impact of the PCMs on reducing the total annual energy demand and energy cost.
Finally, this research proposes a novel process for selecting PCMs as energy efficiency measures for building retrofits. This process facilitates the selection of a building and PCM that are complementary. Implementation of this process has not yet been tested; however, the process was developed based on experimental and simulation results from prior studies, and it would alleviate many of the PCM performance issues documented in those studies.
ContributorsAskari Tari, Neda (Author) / Parrish, Kristen (Thesis advisor) / Bryan, Harvey (Committee member) / Reddy, T. Agami (Committee member) / Arizona State University (Publisher)
Created2020